
Patrick Schramowski
Machine Learning Group, Computer Science Department, TU Darmstadt.
Hochschulstrasse 1, Room S1|03 075, 64289 Darmstadt, Germany
+49 6151 1624413
schramowski@cs.tu-darmstadt.de
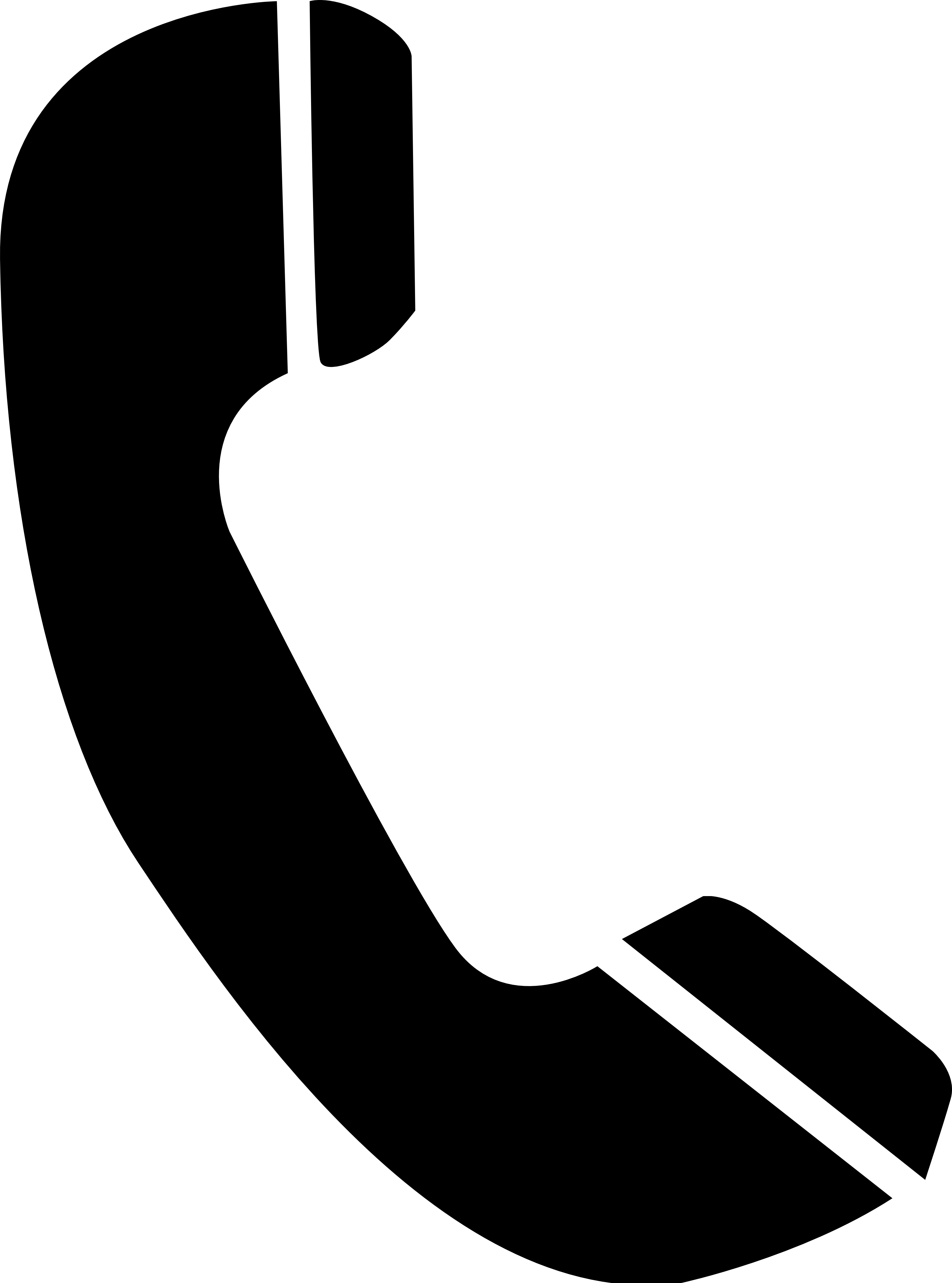
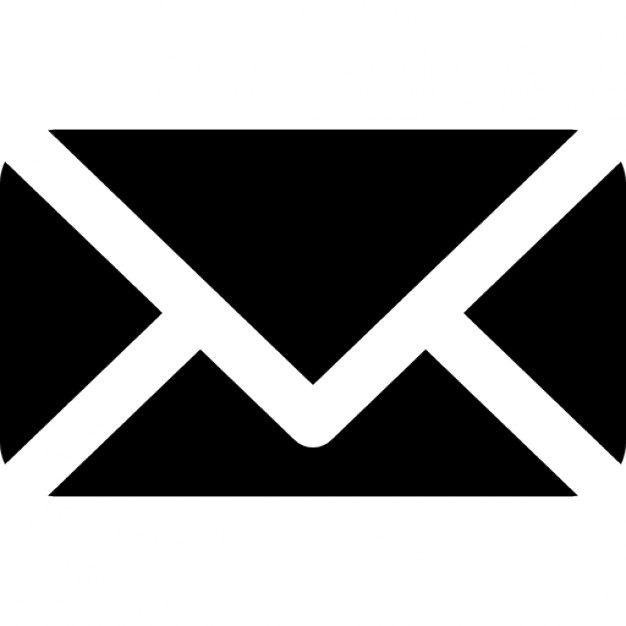
Deutsches Forschungszentrum für KI, TU Darmstadt.
Landwehrstraße 50A, S4 | 23, 1. OG, 64293 Darmstadt, Germany
patrick (dot) schramowski (at) dfki (dot)
de
Landwehrstraße 50A, S4 | 23, 1. OG, 64293 Darmstadt, Germany
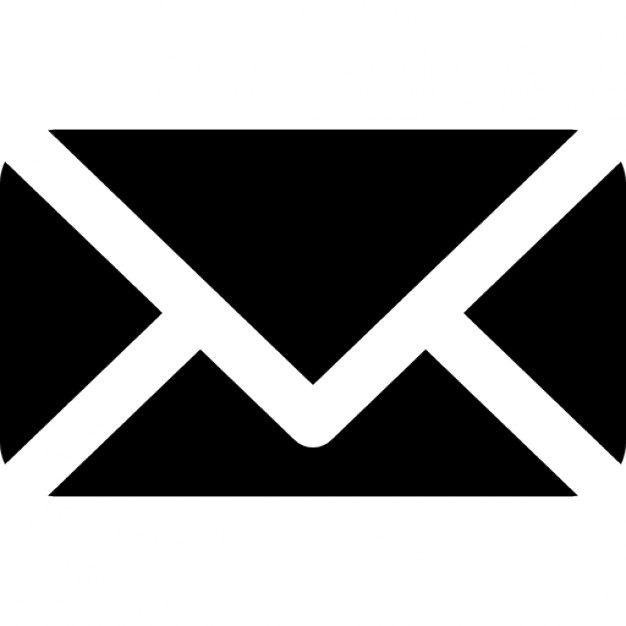
Mission. As AI becomes more advanced, it has the potential to automate many aspects of our lives, but also bring about unintended consequences such as biased decision-making and ethical concerns. My research mission is to explore the ethical implications of AI on society, focusing on large-scale, self-supervised, transformer-based models. I investigate how these models capture hidden knowledge to address ethical concerns. I aim to build human-centric AI systems that mitigate associated risks and solve commonsense tasks.
Bio. I'm a research group leader at the DFKI of the TU Darmstadt University, Germany. After receiving my M.Sc. from the University of Dortmund in 2017, I joined the Machine Learning group in TU Darmstadt University.
Timeline.
2022 - now: | Researcher at DFKI (SAINT) |
2017 - 2023: | Ph.D. student at the Machine Learning Lab, CS Department, TU Darmstadt, Germany |
2016 - 2017: | Co-Founder Pflegix GmbH |
2016 - 2017: | CEO Leanamics UG |
2014 - 2017: | M.Sc. student of computer science at the university of Dortmund, Germany. |
2014 - 2016: | CTO MateApps GmbH |
2011 - 2014: | B.Sc. student of computer science at the university of Dortmund, Germany. |
Publications
Publications can be found at DBLP, SemanticScholar
Publication | Tags |
---|---|
2024 | |
![]() Felix Friedrich, Manuel Brack, Dominik Hintersdorf, Lukas Struppek, Patrick Schramowski, Sasha Luccioni, Kristian Kersting (2024): Auditing and Instructing Text-to-Image Generation Models on Fairness. AI and Ethics.
x pdf bibtex Auditing and Instructing Text-to-Image Generation Models on Fairness Generative AI models have recently achieved astonishing results in quality and are consequently employed in a fast-growing number of applications. However, since they are highly data-driven, relying on billion-sized datasets randomly scraped from the internet, they also suffer from degenerated and biased human behavior, as we demonstrate. In fact, they may even reinforce such biases. To not only uncover but also combat these undesired effects, we present a novel strategy, called Fair Diffusion, to attenuate biases after the deployment of generative text-to-image models. Specifically, we demonstrate shifting a bias, based on human instructions, in any direction yielding arbitrarily new proportions for, e.g., identity groups. As our empirical evaluation demonstrates, this introduced control enables instructing generative image models on fairness, with no data filtering and additional training required. x Auditing and Instructing Text-to-Image Generation Models on Fairness @article{friedrich2024fair, doi = { https://doi.org/10.1007/s43681-024-00531-5 }, publisher = { Springer }, year = { 2024 }, journal = { AI and Ethics }, author = { Felix Friedrich and Manuel Brack and Dominik Hintersdorf and Lukas Struppek and Patrick Schramowski and Sasha Luccioni and Kristian Kersting }, title = { Auditing and Instructing Text-to-Image Generation Models on Fairness } } | Journal AI Ethics, Fairness, Stable Diffusion, Text-Guided Image Generation, Text-to-Image Synthesis |
![]() Dominik Hintersdorf, Lukas Struppek, Manuel Brack, Felix Friedrich, Patrick Schramowski, Kristian Kersting (2024): Does CLIP Know My Face?. Journal of Artificial Intelligence Research (JAIR).
x pdf bibtex Does CLIP Know My Face? With the rise of deep learning in various applications, privacy concerns around the protection of training data has become a critical area of research. Whereas prior studies have focused on privacy risks in single-modal models, we introduce a novel method to assess privacy for multi-modal models, specifically vision-language models like CLIP. The proposed Identity Inference Attack (IDIA) reveals whether an individual was included in the training data by querying the model with images of the same person. Letting the model choose from a wide variety of possible text labels, the model reveals whether it recognizes the person and, therefore, was used for training. Our large-scale experiments on CLIP demonstrate that individuals used for training can be identified with very high accuracy. We confirm that the model has learned to associate names with depicted individuals, implying the existence of sensitive information that can be extracted by adversaries. Our results highlight the need for stronger privacy protection in large-scale models and suggest that IDIAs can be used to prove the unauthorized use of data for training and to enforce privacy laws. x Does CLIP Know My Face? @article{hintersdorf2024clip_privacy, doi = { }, issn = { }, pages = { }, volume = { }, year = { 2024 }, publisher = { }, journal = { Journal of Artificial Intelligence Research (JAIR) }, author = { Dominik Hintersdorf and Lukas Struppek and Manuel Brack and Felix Friedrich and Patrick Schramowski and Kristian Kersting }, title = { Does CLIP Know My Face? } } | Journal CLIP, Computer Vision, Deep Learning, Identity Inference Attacks, Pre-trained models, Privacy |
![]() Hikaru Shindo, Manuel Brack, Gopika Sudhakaran, Devendra Singh Dhami, Patrick Schramowski, Kristian Kersting (2024): DeiSAM: Segment Anything with Deictic Prompting. In Proceedings of the 38th Conference on Neural Information Processing Systems (NeurIPS).
x url bibtex DeiSAM: Segment Anything with Deictic Prompting Large-scale, pre-trained neural networks have demonstrated strong capabilities in various tasks, including zero-shot image segmentation. To identify concrete objects in complex scenes, humans instinctively rely on deictic descriptions in natural language, i.e. , referring to something depending on the con- text, e.g. ”The object that is on the desk and behind the cup.”. However, deep learning approaches cannot reliably interpret these deictic representations due to their lack of reasoning capabilities in complex scenarios. To remedy this issue, we propose DeiSAM, which integrates large pre-trained neural networks with differentiable logic reasoners. Given a complex, textual segmentation description, DeiSAM leverages Large Language Models (LLMs) to generate first-order logic rules and performs differentiable forward reasoning on generated scene graphs. Subsequently, DeiSAM segments objects by matching them to the logically inferred image regions. As part of our evaluation, we propose the Deictic Visual Genome (DeiVG) dataset, containing paired visual input and complex, deictic textual prompts. Our empirical results demonstrate that DeiSAM is a substantial improvement over data-driven neural baselines on deictic segmentation tasks. x DeiSAM: Segment Anything with Deictic Prompting @inproceedings{shindo2024deisam, booktitle = { Proceedings of the 38th Conference on Neural Information Processing Systems (NeurIPS) }, pages = { }, year = { 2024 }, title = { DeiSAM: Segment Anything with Deictic Prompting }, author = { Hikaru Shindo and Manuel Brack and Gopika Sudhakaran and Devendra Singh Dhami and Patrick Schramowski and Kristian Kersting } } | Conference Differentiable Reasoning, Neuro-Symbolic AI, Segmentation, Textual Grounding |
![]() Björn Deiseroth, Manuel Brack, Patrick Schramowski, Kristian Kersting, Samuel Weinbach (2024): T-FREE: Subword Tokenizer-Free Generative LLMs via Sparse Representations for Memory-Efficient Embeddings. In Proceedings of the Conference on Empirical Methods in Natural Language Processing (EMNLP).
x pdf bibtex T-FREE: Subword Tokenizer-Free Generative LLMs via Sparse Representations for Memory-Efficient Embeddings Tokenizers are crucial for encoding information in Large Language Models, but their development has recently stagnated, and they contain inherent weaknesses. Major limitations include computational overhead, ineffective vocabulary use, and unnecessarily large embedding and head layers. Additionally, their performance is biased towards a reference corpus, leading to reduced effectiveness for underrepresented languages. To remedy these issues, we propose T-FREE, which directly embeds words through sparse activation patterns over character triplets, and does not require a reference corpus. T-FREE inherently exploits morphological similarities and allows for strong compression of embedding layers. In our exhaustive experimental evaluation, we achieve competitive downstream performance with a parameter reduction of more than 85% on these layers. Further, T-FREE shows significant improvements in cross-lingual transfer learning. x T-FREE: Subword Tokenizer-Free Generative LLMs via Sparse Representations for Memory-Efficient Embeddings @inproceedings{deiseroth2024emnlp, booktitle = { Proceedings of the Conference on Empirical Methods in Natural Language Processing (EMNLP) }, year = { 2024 }, author = { Björn Deiseroth and Manuel Brack and Patrick Schramowski and Kristian Kersting and Samuel Weinbach }, title = { T-FREE: Subword Tokenizer-Free Generative LLMs via Sparse Representations for Memory-Efficient Embeddings } } | Conference Large Language Models, Memory-Efficient Embeddings, Sparse Representations, Tokenizers |
![]() Quentin Delfosse, Patrick Schramowski, Martin Mundt, Alejandro Molina, Kristian Kersting (2024): Adaptive Rational Activations to Boost Deep Reinforcement Learning. In Proceedings of the International Conference on Representation Learning (ICLR) .
x pdf bibtex Adaptive Rational Activations to Boost Deep Reinforcement Learning Latest insights from biology show that intelligence not only emerges from the connections between neurons, but that individual neurons shoulder more computational responsibility than previously anticipated. Specifically, neural plasticity should be critical in the context of constantly changing reinforcement learning (RL) environments, yet current approaches still primarily employ static activation functions. In this work, we motivate the use of adaptable activation functions in RL and show that rational activation functions are particularly suitable for augmenting plasticity. Inspired by residual networks, we derive a condition under which rational units are closed under residual connections and formulate a naturally regularised version. The proposed joint-rational activation allows for desirable degrees of flexibility, yet regularises plasticity to an extent that avoids overfitting by leveraging a mutual set of activation function parameters across layers. We demonstrate that equipping popular algorithms with (joint) rational activations leads to consistent improvements on different games from the Atari Learning Environment benchmark, notably making DQN competitive to DDQN and Rainbow. x Adaptive Rational Activations to Boost Deep Reinforcement Learning @inproceedings{delfosse2024raRL, year = { 2024 }, author = { Quentin Delfosse and Patrick Schramowski and Martin Mundt and Alejandro Molina and Kristian Kersting }, title = { Adaptive Rational Activations to Boost Deep Reinforcement Learning }, booktitle = { Proceedings of the International Conference on Representation Learning (ICLR) } } | Conference Deep Reinforcement Learning, Neural Plasticity, Rational Activations |
![]() Manuel Brack, Felix Friedrich, Katharina Kornmeier, Linoy Tsaban, Patrick Schramowski, Kristian Kersting, Apolinaros Passos (2024): LEDITS++: Limitless Image Editing using Text-to-Image Models. In Proceedings of the IEEE/CVF Conference on Computer Vision and Pattern Recognition (CVPR).
x pdf bibtex LEDITS++: Limitless Image Editing using Text-to-Image Models Text-to-image diffusion models have recently received a lot of interest for their astonishing ability to produce high-fidelity images from text only. Subsequent research efforts are aiming to exploit the capabilities of these models and leverage them for intuitive, textual image editing. However, existing methods often require time-consuming fine-tuning and lack native support for performing multiple edits simultaneously. To address these issues, we introduce LEDITS++ , an efficient yet versatile technique for image editing using text-to-image models. LEDITS++ requires no tuning nor optimization, runs in a few diffusion steps, natively supports multiple simultaneous edits, inherently limits changes to relevant image regions, and is architecture agnostic. x LEDITS++: Limitless Image Editing using Text-to-Image Models @inproceedings{brack2024ledits, pages = { }, year = { 2024 }, booktitle = { Proceedings of the IEEE/CVF Conference on Computer Vision and Pattern Recognition (CVPR) }, author = { Manuel Brack and Felix Friedrich and Katharina Kornmeier and Linoy Tsaban and Patrick Schramowski and Kristian Kersting and Apolinaros Passos }, title = { LEDITS++: Limitless Image Editing using Text-to-Image Models } } | Conference Image Editing, Semantics, Stable Diffusion, Text-Guided Image Generation, Text-to-Image Synthesis |
![]() Björn Deiseroth, Max Meuer, Nikolas Gritsch, Constantin Eichenberg, Patrick Schramowski, Matthias Aßenmacher, Kristian Kersting (2024): Divergent Token Metrics: Measuring degradation to prune away LLM components – and optimize quantization. In Proceedings of the 2024 Conference of the North American Chapter of the Association for Computational Linguistics (NAACL 2024) .
x pdf bibtex Divergent Token Metrics: Measuring degradation to prune away LLM components – and optimize quantization Large Language Models (LLMs) have reshaped natural language processing with their impressive capabilities. Their ever-increasing size, however, have raised concerns about their effective deployment and the need for LLM compression. This study introduces the Divergent Token Metrics (DTMs), a novel approach for assessing compressed LLMs, addressing the limitations of traditional perplexity or accuracy measures that fail to accurately reflect text generation quality. DTMs focus on token divergence, that allow deeper insights into the subtleties of model compression, in particular when evaluating components' impacts individually. Utilizing the First Divergent Token Metric (FDTM) in model sparsification reveals that 25% of all attention components can be pruned beyond 90% on the Llama-2 model family, still keeping SOTA performance. For quantization FDTM suggests that over 80% of parameters can naively be transformed to int8 without special outlier management. These evaluations indicate the necessity of choosing appropriate compressions for parameters individually---and that FDTM can identify those---while standard metrics result in deteriorated outcomes. x Divergent Token Metrics: Measuring degradation to prune away LLM components – and optimize quantization @inproceedings{deiseroth2024dtm, pages = { }, year = { 2024 }, author = { Björn Deiseroth and Max Meuer and Nikolas Gritsch and Constantin Eichenberg and Patrick Schramowski and Matthias Aßenmacher and Kristian Kersting }, title = { Divergent Token Metrics: Measuring degradation to prune away LLM components – and optimize quantization }, booktitle = { Proceedings of the 2024 Conference of the North American Chapter of the Association for Computational Linguistics (NAACL 2024) } } | Conference Deep Learning, Efficiency, Interpretability, Low Compute Setting, Model Analysdis, Quantization |
![]() Lukas Helff, Felix Friedrich, Manuel Brack, Patrick Schramowski, Kristian Kersting (2024): LLAVAGUARD: VLM-based Safeguard for Vision Dataset Curation and Safety Assessment. In Best Runner-Up Award at NeurIPS RBFM workshop, preprint at arxiv:2406.05113.
x url bibtex LLAVAGUARD: VLM-based Safeguard for Vision Dataset Curation and Safety Assessment We introduce LlavaGuard, a family of multimodal safeguard models based on Llava, offering a robust framework for evaluating the safety compliance of vision datasets and models. Our models come with a new taxonomy designed for assessing safety risks within visual data. With this safety taxonomy, we have collected and annotated a high-quality dataset to guide Vision-Language Models (VLMs) in safety. We present models in two sizes, namely LlavaGuard-7b and LlavaGuard-13b, both safety-tuned on our novel, annotated dataset to perform policy-based safety assessments of visual content. In this context, LlavaGuard goes beyond binary safety classification by providing information on the violated safety categories, a detailed explanation, and a final assessment. In our evaluations, our models demonstrate state-of-the-art performance with LlavaGuard-13b exhibiting the best results, while the much smaller LlavaGuard-7b model outperforms the much larger Llava-34b baseline. Furthermore, LlavaGuard is designed to allow for customization of the safety taxonomy to align with specific use cases, facilitating zero-shot prompting with individual policies for tailored content moderation x LLAVAGUARD: VLM-based Safeguard for Vision Dataset Curation and Safety Assessment @incollection{helff2024llavaguard, booktitle = { Best Runner-Up Award at NeurIPS RBFM workshop, preprint at arxiv:2406.05113 }, year = { 2024 }, author = { Lukas Helff and Felix Friedrich and Manuel Brack and Patrick Schramowski and Kristian Kersting }, title = { LLAVAGUARD: VLM-based Safeguard for Vision Dataset Curation and Safety Assessment } } | Collection AI Safety, Multimodal, Safety Evaluation, Vision Language Model |
![]() Lukas Struppek, Dominik Hintersdorf, Felix Friedrich, Manuel Brack, Patrick Schramowski, Kristian Kersting (2024): Exploiting Cultural Biases via Homoglyphs in Text-to-Image Synthesis. In ICLR 2024 Workshop on Navigating and Addressing Data Problems for Foundation Models (DPFM).
x pdf bibtex Exploiting Cultural Biases via Homoglyphs in Text-to-Image Synthesis Models for text-to-image synthesis have recently drawn a lot of interest. They are capable of producing high-quality images that depict a variety of concepts and styles when conditioned on textual descriptions. However, these models adopt cultural characteristics associated with specific Unicode scripts from their vast amount of training data, which may not be immediately apparent. We show that by simply inserting single non-Latin characters in the textual description, common models reflect cultural biases in their generated images. We analyze this behavior both qualitatively and quantitatively, and identify a model's text encoder as the root cause of the phenomenon. Such behavior can be interpreted as a model feature, offering users a simple way to customize the image generation and reflect their own cultural background. Yet, malicious users or service providers may also try to intentionally bias the image generation. One goal might be to create racist stereotypes by replacing Latin characters with similarly-looking characters from non-Latin scripts, so-called homoglyphs. x Best Paper Award at DPFM 2024 Exploiting Cultural Biases via Homoglyphs in Text-to-Image Synthesis @incollection{struppek2024homoglyphs, year = { 2024 }, crossref = { }, key = { Best Paper Award at DPFM 2024 }, title = { Exploiting Cultural Biases via Homoglyphs in Text-to-Image Synthesis }, pages = { }, booktitle = { ICLR 2024 Workshop on Navigating and Addressing Data Problems for Foundation Models (DPFM) }, author = { Lukas Struppek and Dominik Hintersdorf and Felix Friedrich and Manuel Brack and Patrick Schramowski and Kristian Kersting } } | Collection Cultural biases, Generative AI, Multimodal Systems, Text-guided image generation, Text-to-image synthesis |
![]() Hikaru Shindo, Manuel Brack, Gopika Sudhakaran, Devendra Singh Dhami, Patrick Schramowski, Kristian Kersting (2024): DeiSAM: Segment Anything with Deictic Prompting. In AAAI 2024 Workshop on Neuro-Symbolic Learning and Reasoning
in the Era of Large Language Models (NucLeaR).
x url bibtex DeiSAM: Segment Anything with Deictic Prompting Large-scale, pre-trained neural networks have demonstrated strong capabilities in various tasks, including zero-shot image segmentation. To identify concrete objects in complex scenes, humans instinctively rely on deictic descriptions in natural language, i.e. , referring to something depending on the context, e.g. ”The object that is on the desk and behind the cup.”. However, deep learning approaches cannot reliably interpret these deictic representations due to their lack of reasoning capabilities in complex scenarios. To remedy this issue, we propose DeiSAM, which integrates large pre-trained neural networks with differentiable logic reasoners. Given a complex, textual segmentation description, DeiSAM leverages Large Language Models (LLMs) to generate first-order logic rules and performs differentiable forward reasoning on generated scene graphs. Subsequently, DeiSAM segments objects by matching them to the logically inferred image regions. As part of our evaluation, we propose the Deictic Visual Genome (DeiVG) dataset, containing paired visual input and complex, deictic textual prompts. Our empirical results demonstrate that DeiSAM is a substantial improvement over data-driven neural baselines on deictic segmentation tasks. x DeiSAM: Segment Anything with Deictic Prompting @incollection{shindo2024deisam, booktitle = { AAAI 2024 Workshop on Neuro-Symbolic Learning and Reasoning in the Era of Large Language Models (NucLeaR) }, pages = { }, year = { 2024 }, title = { DeiSAM: Segment Anything with Deictic Prompting }, author = { Hikaru Shindo and Manuel Brack and Gopika Sudhakaran and Devendra Singh Dhami and Patrick Schramowski and Kristian Kersting } } | Collection Differentiable Reasoning, Neuro-Symbolic AI, Segmentation, Textual Grounding |
![]() Felix Friedrich, Simone Tedeschi, Patrick Schramowski, Manuel Brack, Roberto Navigli, Huu Nguyen, Bo Li, Kristian Kersting (2024): LLMs Lost in Translation: M-ALERT uncovers Cross-Linguistic Safety Gaps. arXiv preprint arXiv:2412.15035 .
x url bibtex LLMs Lost in Translation: M-ALERT uncovers Cross-Linguistic Safety Gaps Building safe Large Language Models (LLMs) across multiple languages is essential in ensuring both safe access and linguistic diversity. To this end, we introduce M-ALERT, a multilingual benchmark that evaluates the safety of LLMs in five languages: English, French, German, Italian, and Spanish. M-ALERT includes 15k high-quality prompts per language, totaling 75k, following the detailed ALERT taxonomy. Our extensive experiments on 10 state-of-the-art LLMs highlight the importance of language-specific safety analysis, revealing that models often exhibit significant inconsistencies in safety across languages and categories. For instance, Llama3.2 shows high unsafety in the category crime_tax for Italian but remains safe in other languages. Similar differences can be observed across all models. In contrast, certain categories, such as substance_cannabis and crime_propaganda, consistently trigger unsafe responses across models and languages. These findings underscore the need for robust multilingual safety practices in LLMs to ensure safe and responsible usage across diverse user communities. x LLMs Lost in Translation: M-ALERT uncovers Cross-Linguistic Safety Gaps @misc{friedrich2024llms, howpublished = { arXiv preprint arXiv:2412.15035 }, year = { 2024 }, author = { Felix Friedrich and Simone Tedeschi and Patrick Schramowski and Manuel Brack and Roberto Navigli and Huu Nguyen and Bo Li and Kristian Kersting }, title = { LLMs Lost in Translation: M-ALERT uncovers Cross-Linguistic Safety Gaps } } | Misc AI Safety, Benchmark, Large Language Models, Multilingual, Red Teaming |
![]() Ruben Härle, Felix Friedrich, Manuel Brack, Björn Deiseroth, Patrick Schramowski, Kristian Kersting (2024): SCAR: Sparse Conditioned Autoencoders for Concept Detection and Steering in LLMs. arXiv preprint arXiv:2411.07122 .
x pdf bibtex SCAR: Sparse Conditioned Autoencoders for Concept Detection and Steering in LLMs Large Language Models (LLMs) have demonstrated remarkable capabilities in generating human-like text, but their output may not be aligned with the user or even produce harmful content. This paper presents a novel approach to detect and steer concepts such as toxicity before generation. We introduce the Sparse Conditioned Autoencoder (SCAR), a single trained module that extends the otherwise untouched LLM. SCAR ensures full steerability, towards and away from concepts (e.g., toxic content), without compromising the quality of the model's text generation on standard evaluation benchmarks. We demonstrate the effective application of our approach through a variety of concepts, including toxicity, safety, and writing style alignment. As such, this work establishes a robust framework for controlling LLM generations, ensuring their ethical and safe deployment in real-world applications. x SCAR: Sparse Conditioned Autoencoders for Concept Detection and Steering in LLMs @misc{haerle2024scarsparseconditionedautoencoders, howpublished = { arXiv preprint arXiv:2411.07122 }, year = { 2024 }, author = { Ruben Härle and Felix Friedrich and Manuel Brack and Björn Deiseroth and Patrick Schramowski and Kristian Kersting }, title = { SCAR: Sparse Conditioned Autoencoders for Concept Detection and Steering in LLMs } } | Misc AI Safety, Concept Steering, Large Language Models, Mechanistic Interpretability, SAEs, Sparse Autoencoder |
![]() Manuel Brack, Malte Ostendorff, Pedro Ortiz Suarez, José Javier Saiz, Iñaki Lacunza Castilla, Jorge Palomar-Giner, Patrick Schramowski, Georg Rehm, Marta Villegas, Kristian Kersting (2024): Community OSCAR: A Community Effort for Multilingual Web Data. Technical Report / Preprint .
x pdf bibtex Community OSCAR: A Community Effort for Multilingual Web Data The development of large language models (LLMs) relies heavily on extensive, high-quality datasets. Publicly available datasets focus predominantly on English, leaving other language communities behind. To address this issue, we introduce Community OSCAR, a multilingual dataset initiative designed to address the gap between English and non-English data availability. Through a collective effort, Community OSCAR covers over 150 languages with 45 billion documents, totaling over 345 TiB of data. Initial results indicate that Community OSCAR provides valuable raw data for training LLMs and enhancing the performance of multilingual models. This work aims to contribute to the ongoing advancements in multilingual NLP and to support a more inclusive AI ecosystem by making high-quality, multilingual data more accessible to those working with low-resource languages. x Community OSCAR: A Community Effort for Multilingual Web Data @misc{brack2024communityoscar, howpublished = { Technical Report / Preprint }, year = { 2024 }, author = { Manuel Brack and Malte Ostendorff and Pedro Ortiz Suarez and José Javier Saiz and Iñaki Lacunza Castilla and Jorge Palomar-Giner and Patrick Schramowski and Georg Rehm and Marta Villegas and Kristian Kersting }, title = { Community OSCAR: A Community Effort for Multilingual Web Data } } | Misc Dataset, LLM, LLM training, Large-scale Data, Multilingual |
![]() Manuel Brack, Marlon May, Linoy Tsaban, Felix Friedrich, Patrick Schramowski, Apolinaros Passos, Kristian Kersting (2024): Unleashing Creativity: Generalizing Semantic Control for Text-to-Image Diffusion Models. Technical Report / Preprint .
x pdf bibtex Unleashing Creativity: Generalizing Semantic Control for Text-to-Image Diffusion Models The recent surge in popularity of text-to-image diffusion models (DMs) can largely be attributed to the versatile, expressive, and intuitive user interfaces provided through textual prompts. These models enable inexperienced people to explore artistic ventures easily and provide exciting new opportunities to experienced artists. However, the semantic control offered through text prompts alone is limited and rather fragile, and overall lacks the fine granularity necessary for creative applications. The majority of methods addressing this issue are restricted to specific DM architectures, severely limiting the creative workflow instead of generalizing it to arbitrary models. In contrast, we demonstrate that semantic guidance (SEGA) generalizes to any DM architecture. Importantly, SEGA is natively compatible with state-of-the-art diffusion transformers. Our empirical results show strong model-agnostic performance, and we highlight new creative possibilities enabled by SEGA, such as enhanced typographic manipulations. This work underscores SEGA’s potential to provide consistent, high-quality semantic guidance in a rapidly evolving generative model landscape. x Unleashing Creativity: Generalizing Semantic Control for Text-to-Image Diffusion Models @misc{brack2024unleashing, howpublished = { Technical Report / Preprint }, year = { 2024 }, author = { Manuel Brack and Marlon May and Linoy Tsaban and Felix Friedrich and Patrick Schramowski and Apolinaros Passos and Kristian Kersting }, title = { Unleashing Creativity: Generalizing Semantic Control for Text-to-Image Diffusion Models } } | Misc Diffusion Transformers, SEGA, Semantic Control, Text-Guided Image Generation, Text-to-Image Synthesis |
![]() Björn Deiseroth, Manuel Brack, Patrick Schramowski, Kristian Kersting, Samuel Weinbach (2024): T-FREE: Tokenizer-Free Generative LLMs via Sparse Representations for Memory-Efficient Embeddings. arXiv preprint arXiv:2406.19223 .
x url bibtex T-FREE: Tokenizer-Free Generative LLMs via Sparse Representations for Memory-Efficient Embeddings Tokenizers are crucial for encoding information in Large Language Models, but their development has recently stagnated, and they contain inherent weaknesses. Major limitations include computational overhead, ineffective vocabulary use, and unnecessarily large embedding and head layers. Additionally, their performance is biased towards a reference corpus, leading to reduced effectiveness for underrepresented languages. To remedy these issues, we propose T-FREE, which directly embeds words through sparse activation patterns over character triplets, and does not require a reference corpus. T-FREE inherently exploits morphological similarities and allows for strong compression of embedding layers. In our exhaustive experimental evaluation, we achieve competitive downstream performance with a parameter reduction of more than 85% on these layers. Further, T-FREE shows significant improvements in cross-lingual transfer learning. x T-FREE: Tokenizer-Free Generative LLMs via Sparse Representations for Memory-Efficient Embeddings @misc{deiseroth2024tfree, howpublished = { arXiv preprint arXiv:2406.19223 }, year = { 2024 }, author = { Björn Deiseroth and Manuel Brack and Patrick Schramowski and Kristian Kersting and Samuel Weinbach }, title = { T-FREE: Tokenizer-Free Generative LLMs via Sparse Representations for Memory-Efficient Embeddings } } | Misc Large Language Models, Memory-Efficient Embeddings, Sparse Representations, Tokenizers |
![]() Simone Tedeschi, Felix Friedrich, Patrick Schramowski, Kristian Kersting, Roberto Navigli, Huu Nguyen, Bo Li (2024): ALERT: A Comprehensive Benchmark for Assessing Large Language Models' Safety through Red Teaming. arXiv preprint arXiv:2404.08676 .
x pdf bibtex ALERT: A Comprehensive Benchmark for Assessing Large Language Models' Safety through Red Teaming When building Large Language Models (LLMs), it is paramount to bear safety in mind and protect them with guardrails. Indeed, LLMs should never generate content promoting or normalizing harmful, illegal, or unethical behavior that may contribute to harm to individuals or society. This principle applies to both normal and adversarial use. In response, we introduce ALERT, a large-scale benchmark to assess safety based on a novel fine-grained risk taxonomy. It is designed to evaluate the safety of LLMs through red teaming methodologies and consists of more than 45k instructions categorized using our novel taxonomy. By subjecting LLMs to adversarial testing scenarios, ALERT aims to identify vulnerabilities, inform improvements, and enhance the overall safety of the language models. Furthermore, the fine-grained taxonomy enables researchers to perform an in-depth evaluation that also helps one to assess the alignment with various policies. In our experiments, we extensively evaluate 10 popular open- and closed-source LLMs and demonstrate that many of them still struggle to attain reasonable levels of safety. x ALERT: A Comprehensive Benchmark for Assessing Large Language Models' Safety through Red Teaming @misc{tedeschi2024alert, howpublished = { arXiv preprint arXiv:2404.08676 }, year = { 2024 }, author = { Simone Tedeschi and Felix Friedrich and Patrick Schramowski and Kristian Kersting and Roberto Navigli and Huu Nguyen and Bo Li }, title = { ALERT: A Comprehensive Benchmark for Assessing Large Language Models' Safety through Red Teaming } } | Misc AI Safety, Benchmark, Evaluation, Large Language Model, Red Teaming, Risk Taxonomy |
![]() Felix Friedrich, Katharina Hämmerl, Patrick Schramowski, Manuel Brack, Jindrich Libovicky, Kristian Kersting, Alexander Fraser (2024): Multilingual Text-to-Image Generation Magnifies Gender Stereotypes and Prompt Engineering May Not Help You. arXiv preprint arXiv:2401.16092 .
x pdf bibtex Multilingual Text-to-Image Generation Magnifies Gender Stereotypes and Prompt Engineering May Not Help You Text-to-image generation models have recently achieved astonishing results in image quality, flexibility, and text alignment and are consequently employed in a fast-growing number of applications. Through improvements in multilingual abilities, a larger community now has access to this kind of technology. Yet, as we will show, multilingual models suffer similarly from (gender) biases as monolingual models. Furthermore, the natural expectation is that these models will provide similar results across languages, but this is not the case and there are important differences between languages. Thus, we propose a novel benchmark MAGBIG intending to foster research in multilingual models without gender bias. We investigate whether multilingual T2I models magnify gender bias with MAGBIG. To this end, we use multilingual prompts requesting portrait images of persons of a certain occupation or trait (using adjectives). Our results show not only that models deviate from the normative assumption that each gender should be equally likely to be generated, but that there are also big differences across languages. Furthermore, we investigate prompt engineering strategies, i.e. the use of indirect, neutral formulations, as a possible remedy for these biases. Unfortunately, they help only to a limited extent and result in worse text-to-image alignment. Consequently, this work calls for more research into diverse representations across languages in image generators. x Multilingual Text-to-Image Generation Magnifies Gender Stereotypes and Prompt Engineering May Not Help You @misc{friedrich2024multilingual, pages = { }, year = { 2024 }, howpublished = { arXiv preprint arXiv:2401.16092 }, title = { Multilingual Text-to-Image Generation Magnifies Gender Stereotypes and Prompt Engineering May Not Help You }, author = { Felix Friedrich and Katharina Hämmerl and Patrick Schramowski and Manuel Brack and Jindrich Libovicky and Kristian Kersting and Alexander Fraser } } | Misc AI Ethics, Generative AI, Text-to-Image Models |
2023 | |
![]() Felix Friedrich, Wolfgang Stammer, Patrick Schramowski, Kristian Kersting (2023): A typology for exploring the mitigation of shortcut behaviour. Nature Machine Intelligence 5:319-330.
x url bibtex A typology for exploring the mitigation of shortcut behaviour As machine learning models become larger, and are increasingly trained on large and uncurated datasets in weakly supervised mode, it becomes important to establish mechanisms for inspecting, interacting with and revising models. These are necessary to mitigate shortcut learning effects and to guarantee that the model’s learned knowledge is aligned with human knowledge. Recently, several explanatory interactive machine learning methods have been developed for this purpose, but each has different motivations and methodological details. In this work, we provide a unification of various explanatory interactive machine learning methods into a single typology by establishing a common set of basic modules. We discuss benchmarks and other measures for evaluating the overall abilities of explanatory interactive machine learning methods. With this extensive toolbox, we systematically and quantitatively compare several explanatory interactive machine learning methods. In our evaluations, all methods are shown to improve machine learning models in terms of accuracy and explainability. However, we found remarkable differences in individual benchmark tasks, which reveal valuable application-relevant aspects for the integration of these benchmarks in the development of future methods. x A typology for exploring the mitigation of shortcut behaviour @article{friedrich2023xiltypology, doi = { 10.1038/s42256-023-00612-w }, issn = { 2522-5839 }, pages = { 319-330 }, volume = { 5 }, year = { 2023 }, publisher = { Nature Publishing Group }, journal = { Nature Machine Intelligence }, author = { Felix Friedrich and Wolfgang Stammer and Patrick Schramowski and Kristian Kersting }, title = { A typology for exploring the mitigation of shortcut behaviour } } | Journal Explainable Artificial Intelligence, Explanatory Interactive Machine Learning, Human-AI Interaction, Human-guided AI, Research Comparability, Research Transparency, XAI, XIL |
![]() Lukas Struppek, Dominik Hintersdorf, Felix Friedrich, Manuel Brack, Patrick Schramowski, Kristian Kersting (2023): Exploiting Cultural Biases via Homoglyphs in Text-to-Image Synthesis. Journal of Artificial Intelligence Research (JAIR).
x url bibtex Exploiting Cultural Biases via Homoglyphs in Text-to-Image Synthesis Models for text-to-image synthesis, such as DALL-E 2 and Stable Diffusion, have recently drawn a lot of interest from academia and the general public. These models are capable of producing high-quality images that depict a variety of concepts and styles when conditioned on textual descriptions. However, these models adopt cultural characteristics associated with specific Unicode scripts from their vast amount of training data, which may not be immediately apparent. We show that by simply inserting single non-Latin characters in the textual description, common models reflect cultural biases in their generated images. We analyze this behavior both qualitatively and quantitatively and identify a model’s text encoder as the root cause of the phenomenon. Such behavior can be interpreted as a model feature, offering users a simple way to customize the image generation and reflect their own cultural background. Yet, malicious users or service providers may also try to intentionally bias the image generation. One goal might be to create racist stereotypes by replacing Latin characters with similarly-looking characters from non-Latin scripts, so-called homoglyphs. To mitigate such unnoticed script attacks, we propose a novel homoglyph unlearning method to fine-tune a text encoder, making it robust against homoglyph manipulations x Exploiting Cultural Biases via Homoglyphs in Text-to-Image Synthesis @article{struppek2023jair, year = { 2023 }, crossref = { }, title = { Exploiting Cultural Biases via Homoglyphs in Text-to-Image Synthesis }, pages = { }, volume = { }, journal = { Journal of Artificial Intelligence Research (JAIR) }, author = { Lukas Struppek and Dominik Hintersdorf and Felix Friedrich and Manuel Brack and Patrick Schramowski and Kristian Kersting } } | Journal Cultural biases, Generative AI, Multimodal Systems, Text-guided image generation, Text-to-image synthesis |
![]() Nicolas Pfeuffer, Lorenz Baum, Wolfgang Stammer, Benjamin M. Abdel-Karim, Patrick Schramowski, Andreas M. Bucher, Christian Hügel, Gernot Rohde, Kristian Kersting, Oliver Hinz (2023): Explanatory Interactive Machine Learning: Establishing an Action Design Research Process for Machine Learning Projects. Business & Information Systems Engineering.
x bibtex Explanatory Interactive Machine Learning: Establishing an Action Design Research Process for Machine Learning Projects The most promising standard machine learning methods can deliver highly accurate classification results and often outperform standard white-box methods. However, for humans, it is hardly possible to fully understand the rationale behind the black-box results, and thus, these powerful methods hamper the creation of new knowledge on the part of humans and the acceptance of this technology on a broader basis. Explainable Artificial Intelligence tries to solve this problem by making the results more interpretable, while Interactive Machine Learning integrates humans into the process of insight discovery. We build upon recent successes of combining these two cuttingedge technologies and propose how Explanatory Interactive Machine Learning (XIL) is embedded in a generalizable Action Design Research (ADR) process – which we call XIL-ADR. This approach can be used to analyze data, inspect models, and iteratively improve them. We show the application of this process and use the diagnosis of viral pneumonia, e.g., Covid-19, as an illustrative example. By this means, this paper also illustrates how XIL-ADR can help identify shortcomings of standard machine learning projects, gain new insights on the part of the human user, and thereby help to tap the full potential of AI-based systems for organizations and research. x Explanatory Interactive Machine Learning: Establishing an Action Design Research Process for Machine Learning Projects @article{pfeuffer2023xil, author = { Nicolas Pfeuffer and Lorenz Baum and Wolfgang Stammer and Benjamin M. Abdel-Karim and Patrick Schramowski and Andreas M. Bucher and Christian Hügel and Gernot Rohde and Kristian Kersting and Oliver Hinz }, year = { 2023 }, pages = { }, journal = { Business & Information Systems Engineering }, title = { Explanatory Interactive Machine Learning: Establishing an Action Design Research Process for Machine Learning Projects } } | Journal Action Design Research, COVID-19, Confounders , Explainable AI, Imaging, Interactive Learning, Machine Learning |
![]() Anna Brugger, Facundo Ispizua Yamati, Abel Barreto, Stefan Paulus, Patrick Schramowski, Kristian Kersting, Ulrike Steiner, Susanne Neugart, Anne-Katrin Mahlein (2023): Hyperspectral imaging in the UV-range allows for differentiation of sugar beet diseases based on changes of secondary plant metabolites. Pythopathology 113(1):44-45.
x url bibtex Hyperspectral imaging in the UV-range allows for differentiation of sugar beet diseases based on changes of secondary plant metabolites Fungal infections trigger defense or signaling responses in plants, leading to various changes in plant metabolites. The changes in metabolites, for example chlorophyll or flavonoids, have long been detectable using time-consuming destructive analytical methods including high-performance liquid chromatography or photometric determination. Recent plant phenotyping studies have revealed that hyperspectral imaging (HSI) in the UV-range can be used to link spectral changes with changes in plant metabolites. To compare established destructive analytical methods with new non-destructive hyperspectral measurements, the interaction between sugar beet leaves and the pathogens Cercospora beticola, which causes Cercospora leaf spot disease (CLS), and Uromyces betae, which causes sugar beet rust (BR), was investigated. With the help of destructive analyses, we showed that both diseases have different effects on chlorophylls, carotenoids, flavonoids, and several phenols. Non-destructive hyperspectral measurements in the UV-range revealed different effects of CLS and BR on plant metabolites resulting in distinct reflectance patterns. Both diseases resulted in specific spectral changes that allowed differentiation between the two diseases. Machine learning algorithms enabled the differentiation between the symptom classes and recognition of the two sugar beet diseases. Feature importance analysis identified specific wavelengths important to the classification, highlighting the utility of the UV-range. The study demonstrates that HSI in the UV-range is a promising, non-destructive tool to investigate the influence of plant diseases on plant physiology and biochemistry. x Hyperspectral imaging in the UV-range allows for differentiation of sugar beet diseases based on changes of secondary plant metabolites @article{brugger2022pythopathology, year = { 2023 }, number = { 1 }, isbn = { }, volume = { 113 }, title = { Hyperspectral imaging in the UV-range allows for differentiation of sugar beet diseases based on changes of secondary plant metabolites }, publisher = { APS Publications }, pages = { 44-45 }, journal = { Pythopathology }, author = { Anna Brugger and Facundo Ispizua Yamati and Abel Barreto and Stefan Paulus and Patrick Schramowski and Kristian Kersting and Ulrike Steiner and Susanne Neugart and Anne-Katrin Mahlein } } | Journal HPLC, Hyperspectral imaging, UV-range, machine learning, plant metabolites, sugar beet |
![]() Marco Bellagente, Manuel Brack, Hannah Teufel, Felix Friedrich, Björn Deiseroth, Constantin Eichenberg, Andrew Dai, Robert Baldock, Souradeep Nanda, Koen Oostermeijer, Andres Felipe Cruz-Salinas, Patrick Schramowski, Kristian Kersting, Samuel Weinbach (2023): MultiFusion: Fusing Pre-Trained Models for Multi-Lingual, Multi-Modal Image Generation. In Proceedings of the 37th Conference on Neural Information Processing Systems (NeurIPS).
x pdf bibtex MultiFusion: Fusing Pre-Trained Models for Multi-Lingual, Multi-Modal Image Generation The recent popularity of text-to-image diffusion models (DM) can largely be attributed to the intuitive interface they provide to users. The intended generation can be expressed in natural language, with the model producing faithful interpretations of text prompts. However, expressing complex or nuanced ideas in text alone can be difficult. To ease image generation, we propose MultiFusion that allows one to express complex and nuanced concepts with arbitrarily interleaved inputs of multiple modalities and languages. MutliFusion leverages pre-trained models and aligns them for integration into a cohesive system, thereby avoiding the need for extensive training from scratch. Our experimental results demonstrate the efficient transfer of capabilities from individual modules to the downstream model. Specifically, the fusion of all independent components allows the image generation module to utilize multilingual, interleaved multimodal inputs despite being trained solely on monomodal data in a single language. x MultiFusion: Fusing Pre-Trained Models for Multi-Lingual, Multi-Modal Image Generation @inproceedings{bellagente2023multifusion, booktitle = { Proceedings of the 37th Conference on Neural Information Processing Systems (NeurIPS) }, pages = { }, year = { 2023 }, title = { MultiFusion: Fusing Pre-Trained Models for Multi-Lingual, Multi-Modal Image Generation }, author = { Marco Bellagente and Manuel Brack and Hannah Teufel and Felix Friedrich and Björn Deiseroth and Constantin Eichenberg and Andrew Dai and Robert Baldock and Souradeep Nanda and Koen Oostermeijer and Andres Felipe Cruz-Salinas and Patrick Schramowski and Kristian Kersting and Samuel Weinbach } } | Conference Diffusion, Image Generation, Image Synthesis, Multilingualism, Multimodality |
![]() Björn Deiseroth, Mayukh Deb, Samuel Weinbach, Manuel Brack, Patrick Schramowski, Kristian Kersting (2023): AtMan: Understanding Transformer Predictions Through Memory Efficient Attention Manipulation. In Proceedings of the 37th Conference on Neural Information Processing Systems (NeurIPS).
x pdf bibtex AtMan: Understanding Transformer Predictions Through Memory Efficient Attention Manipulation Generative transformer models have become increasingly complex, with large numbers of parameters and the ability to process multiple input modalities. Current methods for explaining their predictions are resource-intensive. Most crucially, they require prohibitively large amounts of additional memory since they rely on backpropagation which allocates almost twice as much GPU memory as the forward pass. This renders it difficult, if not impossible, to use explanations in production. We present AtMan that provides explanations of generative transformer models at almost no extra cost. Specifically, AtMan is a modality-agnostic perturbation method that manipulates the attention mechanisms of transformers to produce relevance maps for the input with respect to the output prediction. Instead of using backpropagation, AtMan applies a parallelizable token-based search method relying on cosine similarity neighborhood in the embedding space. Our exhaustive experiments on text and image-text benchmarks demonstrate that AtMan outperforms current state-of-the-art gradient-based methods on several metrics and models while being computationally efficient. As such, AtMan is suitable for use in large model inference deployments. x code AtMan: Understanding Transformer Predictions Through Memory Efficient Attention Manipulation @inproceedings{deiseroth2023atman, booktitle = { Proceedings of the 37th Conference on Neural Information Processing Systems (NeurIPS) }, crossref = { https://github.com/Aleph-Alpha/AtMan }, year = { 2023 }, title = { AtMan: Understanding Transformer Predictions Through Memory Efficient Attention Manipulation }, author = { Björn Deiseroth and Mayukh Deb and Samuel Weinbach and Manuel Brack and Patrick Schramowski and Kristian Kersting } } | Conference Computer Vision, Explainable AI, Large Language Models, Multimodal, Transformer |
![]() Manuel Brack, Felix Friedrich, Dominik Hintersdorf, Lukas Struppek, Patrick Schramowski, Kristian Kersting (2023): SEGA: Instructing Text-to-Image Models using Semantic Guidance. In Proceedings of the 37th Conference on Neural Information Processing Systems (NeurIPS).
x pdf bibtex SEGA: Instructing Text-to-Image Models using Semantic Guidance Text-to-image diffusion models have recently received a lot of interest for their astonishing ability to produce high-fidelity images from text only. However, achieving one-shot generation that aligns with the user’s intent is nearly impossible, yet small changes to the input prompt often result in very different images. This leaves the user with little semantic control. To put the user in control, we show how to interact with the diffusion process to flexibly steer it along semantic directions. This semantic guidance (SEGA) generalizes to any generative architecture using classifier-free guidance. More importantly, it allows for subtle and extensive edits, composition and style changes, and optimizing the overall artistic conception. We demonstrate SEGA’s effectiveness on both latent and pixel-based diffusion models such as Stable Diffusion, Paella, and DeepFloyd-IF using a variety of tasks, thus providing strong evidence for its versatility and flexibility. x SEGA: Instructing Text-to-Image Models using Semantic Guidance @inproceedings{brack2023sega, booktitle = { Proceedings of the 37th Conference on Neural Information Processing Systems (NeurIPS) }, pages = { }, month = { Dez }, year = { 2023 }, author = { Manuel Brack and Felix Friedrich and Dominik Hintersdorf and Lukas Struppek and Patrick Schramowski and Kristian Kersting }, title = { SEGA: Instructing Text-to-Image Models using Semantic Guidance } } | Conference Concepts, Representations, Semantics, Stable Diffusion, Text-Guided Image Generation, Text-to-Image Synthesis |
![]() Manuel Brack, Patrick Schramowski, Björn Deiseroth, Kristian Kersting (2023): ILLUME: Rationalizing Vision-Language Models through Human Interactions. In Proceedings of the 40th International Conference on Machine Learning (ICML).
x pdf bibtex ILLUME: Rationalizing Vision-Language Models through Human Interactions Bootstrapping from pre-trained language models has been proven to be an efficient approach for building vision-language models (VLM) for tasks such as image captioning or visual question answering. However, outputs of these models rarely align with user's rationales for specific answers. In order to improve this alignment and reinforce commonsense reasons, we propose a tuning paradigm based on human interactions with machine generated data. Our ILLUME executes the following loop: Given an image-question-answer prompt, the VLM samples multiple candidate rationales, and a human critic provides minimal feedback via preference selection, used for fine-tuning. This loop increases the training data and gradually carves out the VLM's rationalization capabilities that are aligned with human intend. Our exhaustive experiments demonstrate that ILLUME is competitive with standard supervised fine-tuning while using significantly fewer training data and only requiring minimal feedback. x ILLUME: Rationalizing Vision-Language Models through Human Interactions @inproceedings{brack2023illume, booktitle = { Proceedings of the 40th International Conference on Machine Learning (ICML) }, month = { Jul }, year = { 2023 }, title = { ILLUME: Rationalizing Vision-Language Models through Human Interactions }, author = { Manuel Brack and Patrick Schramowski and Björn Deiseroth and Kristian Kersting } } | Conference Alignement, Explanatory Interactive Learning, Self-Generated Explanations, XAI |
Showing 1 to 25 of 62 entries
@misc{friedrich2024llms, Anote={../../images/tedeschi2024alert.png}, title={LLMs Lost in Translation: M-ALERT uncovers Cross-Linguistic Safety Gaps}, author={Felix Friedrich and Simone Tedeschi and Patrick Schramowski and Manuel Brack and Roberto Navigli and Huu Nguyen and Bo Li and Kristian Kersting}, year={2024}, Howpublished={arXiv preprint arXiv:2412.15035}, url={https://arxiv.org/abs/2412.15035}, Note = {Building safe Large Language Models (LLMs) across multiple languages is essential in ensuring both safe access and linguistic diversity. To this end, we introduce M-ALERT, a multilingual benchmark that evaluates the safety of LLMs in five languages: English, French, German, Italian, and Spanish. M-ALERT includes 15k high-quality prompts per language, totaling 75k, following the detailed ALERT taxonomy. Our extensive experiments on 10 state-of-the-art LLMs highlight the importance of language-specific safety analysis, revealing that models often exhibit significant inconsistencies in safety across languages and categories. For instance, Llama3.2 shows high unsafety in the category crime_tax for Italian but remains safe in other languages. Similar differences can be observed across all models. In contrast, certain categories, such as substance_cannabis and crime_propaganda, consistently trigger unsafe responses across models and languages. These findings underscore the need for robust multilingual safety practices in LLMs to ensure safe and responsible usage across diverse user communities.}, Keywords = {Multilingual, Red Teaming, Large Language Models, AI Safety, Benchmark} } ,@misc{haerle2024scarsparseconditionedautoencoders, anote={../../images/haerle2024scar.png}, title={SCAR: Sparse Conditioned Autoencoders for Concept Detection and Steering in LLMs}, author={Ruben Härle and Felix Friedrich and Manuel Brack and Björn Deiseroth and Patrick Schramowski and Kristian Kersting}, year={2024}, Howpublished={arXiv preprint arXiv:2411.07122}, url={https://arxiv.org/pdf/2411.07122}, Keywords = {Large Language Models, Concept Steering, Sparse Autoencoder, AI Safety, SAEs, Mechanistic Interpretability}, Note = {Large Language Models (LLMs) have demonstrated remarkable capabilities in generating human-like text, but their output may not be aligned with the user or even produce harmful content. This paper presents a novel approach to detect and steer concepts such as toxicity before generation. We introduce the Sparse Conditioned Autoencoder (SCAR), a single trained module that extends the otherwise untouched LLM. SCAR ensures full steerability, towards and away from concepts (e.g., toxic content), without compromising the quality of the model's text generation on standard evaluation benchmarks. We demonstrate the effective application of our approach through a variety of concepts, including toxicity, safety, and writing style alignment. As such, this work establishes a robust framework for controlling LLM generations, ensuring their ethical and safe deployment in real-world applications.} } ,@inproceedings{shindo2024deisam, Anote={../../images/shindo2024deisam.png}, author = {Hikaru Shindo and Manuel Brack and Gopika Sudhakaran and Devendra Singh Dhami and Patrick Schramowski and Kristian Kersting}, title = {DeiSAM: Segment Anything with Deictic Prompting}, year = {2024}, Url = {https://arxiv.org/abs/2402.14123}, Pages = {}, booktitle = {Proceedings of the 38th Conference on Neural Information Processing Systems (NeurIPS)}, Note = {Large-scale, pre-trained neural networks have demonstrated strong capabilities in various tasks, including zero-shot image segmentation. To identify concrete objects in complex scenes, humans instinctively rely on deictic descriptions in natural language, i.e. , referring to something depending on the con- text, e.g. ”The object that is on the desk and behind the cup.”. However, deep learning approaches cannot reliably interpret these deictic representations due to their lack of reasoning capabilities in complex scenarios. To remedy this issue, we propose DeiSAM, which integrates large pre-trained neural networks with differentiable logic reasoners. Given a complex, textual segmentation description, DeiSAM leverages Large Language Models (LLMs) to generate first-order logic rules and performs differentiable forward reasoning on generated scene graphs. Subsequently, DeiSAM segments objects by matching them to the logically inferred image regions. As part of our evaluation, we propose the Deictic Visual Genome (DeiVG) dataset, containing paired visual input and complex, deictic textual prompts. Our empirical results demonstrate that DeiSAM is a substantial improvement over data-driven neural baselines on deictic segmentation tasks.}, Keywords = {Neuro-Symbolic AI, Differentiable Reasoning, Segmentation, Textual Grounding} } ,@inproceedings{deiseroth2024emnlp, title={T-FREE: Subword Tokenizer-Free Generative LLMs via Sparse Representations for Memory-Efficient Embeddings}, author={Björn Deiseroth and Manuel Brack and Patrick Schramowski and Kristian Kersting and Samuel Weinbach}, year={2024}, booktitle = {Proceedings of the Conference on Empirical Methods in Natural Language Processing (EMNLP)}, Keywords={Large Language Models, Tokenizers, Sparse Representations, Memory-Efficient Embeddings}, Note={Tokenizers are crucial for encoding information in Large Language Models, but their development has recently stagnated, and they contain inherent weaknesses. Major limitations include computational overhead, ineffective vocabulary use, and unnecessarily large embedding and head layers. Additionally, their performance is biased towards a reference corpus, leading to reduced effectiveness for underrepresented languages. To remedy these issues, we propose T-FREE, which directly embeds words through sparse activation patterns over character triplets, and does not require a reference corpus. T-FREE inherently exploits morphological similarities and allows for strong compression of embedding layers. In our exhaustive experimental evaluation, we achieve competitive downstream performance with a parameter reduction of more than 85% on these layers. Further, T-FREE shows significant improvements in cross-lingual transfer learning.}, Anote={../../images/deiseroth2024tfree.png}, url={../../papers/deiseroth2024emnlp.pdf} } ,@misc{brack2024communityoscar, title={Community OSCAR: A Community Effort for Multilingual Web Data}, author={Manuel Brack and Malte Ostendorff and Pedro Ortiz Suarez and José Javier Saiz and Iñaki Lacunza Castilla and Jorge Palomar-Giner and Patrick Schramowski and Georg Rehm and Marta Villegas and Kristian Kersting}, year={2024}, Howpublished={Technical Report / Preprint}, Keywords={Large-scale Data, Dataset, LLM training, LLM, Multilingual}, Note={The development of large language models (LLMs) relies heavily on extensive, high-quality datasets. Publicly available datasets focus predominantly on English, leaving other language communities behind. To address this issue, we introduce Community OSCAR, a multilingual dataset initiative designed to address the gap between English and non-English data availability. Through a collective effort, Community OSCAR covers over 150 languages with 45 billion documents, totaling over 345 TiB of data. Initial results indicate that Community OSCAR provides valuable raw data for training LLMs and enhancing the performance of multilingual models. This work aims to contribute to the ongoing advancements in multilingual NLP and to support a more inclusive AI ecosystem by making high-quality, multilingual data more accessible to those working with low-resource languages.}, Anote={../../images/brack2024communityoscar.png}, url={https://occiglot.eu/papers/Community_Oscar.pdf} } ,@misc{brack2024unleashing, title={Unleashing Creativity: Generalizing Semantic Control for Text-to-Image Diffusion Models}, author={Manuel Brack and Marlon May and Linoy Tsaban and Felix Friedrich and Patrick Schramowski and Apolinaros Passos and Kristian Kersting }, year={2024}, Howpublished={Technical Report / Preprint}, Keywords={Text-to-Image Synthesis, Text-Guided Image Generation, SEGA, Semantic Control, Diffusion Transformers}, Note={The recent surge in popularity of text-to-image diffusion models (DMs) can largely be attributed to the versatile, expressive, and intuitive user interfaces provided through textual prompts. These models enable inexperienced people to explore artistic ventures easily and provide exciting new opportunities to experienced artists. However, the semantic control offered through text prompts alone is limited and rather fragile, and overall lacks the fine granularity necessary for creative applications. The majority of methods addressing this issue are restricted to specific DM architectures, severely limiting the creative workflow instead of generalizing it to arbitrary models. In contrast, we demonstrate that semantic guidance (SEGA) generalizes to any DM architecture. Importantly, SEGA is natively compatible with state-of-the-art diffusion transformers. Our empirical results show strong model-agnostic performance, and we highlight new creative possibilities enabled by SEGA, such as enhanced typographic manipulations. This work underscores SEGA’s potential to provide consistent, high-quality semantic guidance in a rapidly evolving generative model landscape.}, Anote={../../images/brack2024unleashing.png}, url={https://www.aiml.informatik.tu-darmstadt.de/papers/brack2024unleashing.pdf}}, } ,@misc{deiseroth2024tfree, title={T-FREE: Tokenizer-Free Generative LLMs via Sparse Representations for Memory-Efficient Embeddings}, author={Björn Deiseroth and Manuel Brack and Patrick Schramowski and Kristian Kersting and Samuel Weinbach}, year={2024}, Howpublished={arXiv preprint arXiv:2406.19223}, Keywords={Large Language Models, Tokenizers, Sparse Representations, Memory-Efficient Embeddings}, Note={Tokenizers are crucial for encoding information in Large Language Models, but their development has recently stagnated, and they contain inherent weaknesses. Major limitations include computational overhead, ineffective vocabulary use, and unnecessarily large embedding and head layers. Additionally, their performance is biased towards a reference corpus, leading to reduced effectiveness for underrepresented languages. To remedy these issues, we propose T-FREE, which directly embeds words through sparse activation patterns over character triplets, and does not require a reference corpus. T-FREE inherently exploits morphological similarities and allows for strong compression of embedding layers. In our exhaustive experimental evaluation, we achieve competitive downstream performance with a parameter reduction of more than 85% on these layers. Further, T-FREE shows significant improvements in cross-lingual transfer learning.}, Anote={../../images/deiseroth2024tfree.png}, url={https://arxiv.org/abs/2406.19223}, } ,@article{friedrich2024fair, Anote = {../../images/ffriedrich_fair_2023.png}, title={Auditing and Instructing Text-to-Image Generation Models on Fairness}, author={Felix Friedrich and Manuel Brack and Dominik Hintersdorf and Lukas Struppek and Patrick Schramowski and Sasha Luccioni and Kristian Kersting}, Journal = {AI and Ethics}, year = {2024}, Note = {Generative AI models have recently achieved astonishing results in quality and are consequently employed in a fast-growing number of applications. However, since they are highly data-driven, relying on billion-sized datasets randomly scraped from the internet, they also suffer from degenerated and biased human behavior, as we demonstrate. In fact, they may even reinforce such biases. To not only uncover but also combat these undesired effects, we present a novel strategy, called Fair Diffusion, to attenuate biases after the deployment of generative text-to-image models. Specifically, we demonstrate shifting a bias, based on human instructions, in any direction yielding arbitrarily new proportions for, e.g., identity groups. As our empirical evaluation demonstrates, this introduced control enables instructing generative image models on fairness, with no data filtering and additional training required.}, Publisher = {Springer}, Keywords = {Fairness, Text-to-Image Synthesis, Text-Guided Image Generation, Stable Diffusion, AI Ethics}, Url={https://link.springer.com/content/pdf/10.1007/s43681-024-00531-5.pdf}, doi={https://doi.org/10.1007/s43681-024-00531-5} } ,@inproceedings{delfosse2024raRL, booktitle = {Proceedings of the International Conference on Representation Learning (ICLR) }, title={Adaptive Rational Activations to Boost Deep Reinforcement Learning}, author={Quentin Delfosse and Patrick Schramowski and Martin Mundt and Alejandro Molina and Kristian Kersting}, year={2024}, Keywords={Neural Plasticity, Deep Reinforcement Learning, Rational Activations}, Anote={../../images/delfosse2024ratRL.png}, Note={Latest insights from biology show that intelligence not only emerges from the connections between neurons, but that individual neurons shoulder more computational responsibility than previously anticipated. Specifically, neural plasticity should be critical in the context of constantly changing reinforcement learning (RL) environments, yet current approaches still primarily employ static activation functions. In this work, we motivate the use of adaptable activation functions in RL and show that rational activation functions are particularly suitable for augmenting plasticity. Inspired by residual networks, we derive a condition under which rational units are closed under residual connections and formulate a naturally regularised version. The proposed joint-rational activation allows for desirable degrees of flexibility, yet regularises plasticity to an extent that avoids overfitting by leveraging a mutual set of activation function parameters across layers. We demonstrate that equipping popular algorithms with (joint) rational activations leads to consistent improvements on different games from the Atari Learning Environment benchmark, notably making DQN competitive to DDQN and Rainbow.}, Url={https://openreview.net/pdf?id=g90ysX1sVs} } ,@inproceedings{brack2024ledits, Anote = {../../images/mbrack_ledits_pp.png}, title={LEDITS++: Limitless Image Editing using Text-to-Image Models}, author={Manuel Brack and Felix Friedrich and Katharina Kornmeier and Linoy Tsaban and Patrick Schramowski and Kristian Kersting and Apolinaros Passos}, booktitle = {Proceedings of the IEEE/CVF Conference on Computer Vision and Pattern Recognition (CVPR)}, year = {2024}, Note = {Text-to-image diffusion models have recently received a lot of interest for their astonishing ability to produce high-fidelity images from text only. Subsequent research efforts are aiming to exploit the capabilities of these models and leverage them for intuitive, textual image editing. However, existing methods often require time-consuming fine-tuning and lack native support for performing multiple edits simultaneously. To address these issues, we introduce LEDITS++ , an efficient yet versatile technique for image editing using text-to-image models. LEDITS++ requires no tuning nor optimization, runs in a few diffusion steps, natively supports multiple simultaneous edits, inherently limits changes to relevant image regions, and is architecture agnostic.}, Pages = {}, Keywords = {Image Editing, Text-to-Image Synthesis, Text-Guided Image Generation, Stable Diffusion, Semantics}, Url={https://openreview.net/pdf?id=bPiTOXLRRQ} } ,@incollection{helff2024llavaguard, Anote={../../images/llavaguard_pipe.png}, title={LLAVAGUARD: VLM-based Safeguard for Vision Dataset Curation and Safety Assessment}, author={Lukas Helff and Felix Friedrich and Manuel Brack and Patrick Schramowski and Kristian Kersting}, year={2024}, booktitle={Best Runner-Up Award at NeurIPS RBFM workshop, preprint at arxiv:2406.05113}, url={https://arxiv.org/abs/2406.05113}, Note = {We introduce LlavaGuard, a family of multimodal safeguard models based on Llava, offering a robust framework for evaluating the safety compliance of vision datasets and models. Our models come with a new taxonomy designed for assessing safety risks within visual data. With this safety taxonomy, we have collected and annotated a high-quality dataset to guide Vision-Language Models (VLMs) in safety. We present models in two sizes, namely LlavaGuard-7b and LlavaGuard-13b, both safety-tuned on our novel, annotated dataset to perform policy-based safety assessments of visual content. In this context, LlavaGuard goes beyond binary safety classification by providing information on the violated safety categories, a detailed explanation, and a final assessment. In our evaluations, our models demonstrate state-of-the-art performance with LlavaGuard-13b exhibiting the best results, while the much smaller LlavaGuard-7b model outperforms the much larger Llava-34b baseline. Furthermore, LlavaGuard is designed to allow for customization of the safety taxonomy to align with specific use cases, facilitating zero-shot prompting with individual policies for tailored content moderation}, Keywords = {AI Safety, Safety Evaluation, Multimodal, Vision Language Model} } ,@misc{tedeschi2024alert, Anote={../../images/tedeschi2024alert.png}, title={ALERT: A Comprehensive Benchmark for Assessing Large Language Models' Safety through Red Teaming}, author={Simone Tedeschi and Felix Friedrich and Patrick Schramowski and Kristian Kersting and Roberto Navigli and Huu Nguyen and Bo Li}, year={2024}, Howpublished={arXiv preprint arXiv:2404.08676}, url={https://arxiv.org/pdf/2404.08676}, Note = {When building Large Language Models (LLMs), it is paramount to bear safety in mind and protect them with guardrails. Indeed, LLMs should never generate content promoting or normalizing harmful, illegal, or unethical behavior that may contribute to harm to individuals or society. This principle applies to both normal and adversarial use. In response, we introduce ALERT, a large-scale benchmark to assess safety based on a novel fine-grained risk taxonomy. It is designed to evaluate the safety of LLMs through red teaming methodologies and consists of more than 45k instructions categorized using our novel taxonomy. By subjecting LLMs to adversarial testing scenarios, ALERT aims to identify vulnerabilities, inform improvements, and enhance the overall safety of the language models. Furthermore, the fine-grained taxonomy enables researchers to perform an in-depth evaluation that also helps one to assess the alignment with various policies. In our experiments, we extensively evaluate 10 popular open- and closed-source LLMs and demonstrate that many of them still struggle to attain reasonable levels of safety.}, Keywords = {Red Teaming, Large Language Model, AI Safety, Benchmark, Evaluation, Risk Taxonomy} } ,@inproceedings{deiseroth2024dtm, Anote = {../../images/deiseroth2024dtm.png}, booktitle = {Proceedings of the 2024 Conference of the North American Chapter of the Association for Computational Linguistics (NAACL 2024) }, title={Divergent Token Metrics: Measuring degradation to prune away LLM components – and optimize quantization}, author={Björn Deiseroth and Max Meuer and Nikolas Gritsch and Constantin Eichenberg and Patrick Schramowski and Matthias Aßenmacher and Kristian Kersting}, Note = {Large Language Models (LLMs) have reshaped natural language processing with their impressive capabilities. Their ever-increasing size, however, have raised concerns about their effective deployment and the need for LLM compression. This study introduces the Divergent Token Metrics (DTMs), a novel approach for assessing compressed LLMs, addressing the limitations of traditional perplexity or accuracy measures that fail to accurately reflect text generation quality. DTMs focus on token divergence, that allow deeper insights into the subtleties of model compression, in particular when evaluating components' impacts individually. Utilizing the First Divergent Token Metric (FDTM) in model sparsification reveals that 25% of all attention components can be pruned beyond 90% on the Llama-2 model family, still keeping SOTA performance. For quantization FDTM suggests that over 80% of parameters can naively be transformed to int8 without special outlier management. These evaluations indicate the necessity of choosing appropriate compressions for parameters individually---and that FDTM can identify those---while standard metrics result in deteriorated outcomes.}, year={2024}, Pages = {}, Keywords = {Quantization, Model Analysdis, Interpretability, Low Compute Setting, Efficiency, Deep Learning}, Url={https://arxiv.org/pdf/2311.01544} } ,@incollection{struppek2024homoglyphs, Anote = {../../images/struppek2023jair.png}, Author = {Lukas Struppek and Dominik Hintersdorf and Felix Friedrich and Manuel Brack and Patrick Schramowski and Kristian Kersting}, booktitle={ICLR 2024 Workshop on Navigating and Addressing Data Problems for Foundation Models (DPFM)}, Keywords = {Generative AI, Text-guided image generation, Text-to-image synthesis, Multimodal Systems, Cultural biases}, Pages = {}, Note = {Models for text-to-image synthesis have recently drawn a lot of interest. They are capable of producing high-quality images that depict a variety of concepts and styles when conditioned on textual descriptions. However, these models adopt cultural characteristics associated with specific Unicode scripts from their vast amount of training data, which may not be immediately apparent. We show that by simply inserting single non-Latin characters in the textual description, common models reflect cultural biases in their generated images. We analyze this behavior both qualitatively and quantitatively, and identify a model's text encoder as the root cause of the phenomenon. Such behavior can be interpreted as a model feature, offering users a simple way to customize the image generation and reflect their own cultural background. Yet, malicious users or service providers may also try to intentionally bias the image generation. One goal might be to create racist stereotypes by replacing Latin characters with similarly-looking characters from non-Latin scripts, so-called homoglyphs.}, Title = {Exploiting Cultural Biases via Homoglyphs in Text-to-Image Synthesis}, Url = {https://openreview.net/pdf?id=VeCTgo5f9q}, Key = {Best Paper Award at DPFM 2024}, Crossref = {}, Year = {2024} } ,@misc{friedrich2024multilingual, Anote={../../images/magbig.png}, author = {Felix Friedrich and Katharina Hämmerl and Patrick Schramowski and Manuel Brack and Jindrich Libovicky and Kristian Kersting and Alexander Fraser}, title={Multilingual Text-to-Image Generation Magnifies Gender Stereotypes and Prompt Engineering May Not Help You}, Howpublished = {arXiv preprint arXiv:2401.16092}, year = {2024}, Url = {https://arxiv.org/pdf/2401.16092}, Pages = {}, Note = {Text-to-image generation models have recently achieved astonishing results in image quality, flexibility, and text alignment and are consequently employed in a fast-growing number of applications. Through improvements in multilingual abilities, a larger community now has access to this kind of technology. Yet, as we will show, multilingual models suffer similarly from (gender) biases as monolingual models. Furthermore, the natural expectation is that these models will provide similar results across languages, but this is not the case and there are important differences between languages. Thus, we propose a novel benchmark MAGBIG intending to foster research in multilingual models without gender bias. We investigate whether multilingual T2I models magnify gender bias with MAGBIG. To this end, we use multilingual prompts requesting portrait images of persons of a certain occupation or trait (using adjectives). Our results show not only that models deviate from the normative assumption that each gender should be equally likely to be generated, but that there are also big differences across languages. Furthermore, we investigate prompt engineering strategies, i.e. the use of indirect, neutral formulations, as a possible remedy for these biases. Unfortunately, they help only to a limited extent and result in worse text-to-image alignment. Consequently, this work calls for more research into diverse representations across languages in image generators.}, Keywords = {AI Ethics, Generative AI, Text-to-Image Models} } ,@article{hintersdorf2024clip_privacy, Anote = {../../images/hintersdorf2022clipping_privacy.png}, title = {Does CLIP Know My Face?}, author={Dominik Hintersdorf and Lukas Struppek and Manuel Brack and Felix Friedrich and Patrick Schramowski and Kristian Kersting}, Journal = {Journal of Artificial Intelligence Research (JAIR)}, Note = {With the rise of deep learning in various applications, privacy concerns around the protection of training data has become a critical area of research. Whereas prior studies have focused on privacy risks in single-modal models, we introduce a novel method to assess privacy for multi-modal models, specifically vision-language models like CLIP. The proposed Identity Inference Attack (IDIA) reveals whether an individual was included in the training data by querying the model with images of the same person. Letting the model choose from a wide variety of possible text labels, the model reveals whether it recognizes the person and, therefore, was used for training. Our large-scale experiments on CLIP demonstrate that individuals used for training can be identified with very high accuracy. We confirm that the model has learned to associate names with depicted individuals, implying the existence of sensitive information that can be extracted by adversaries. Our results highlight the need for stronger privacy protection in large-scale models and suggest that IDIAs can be used to prove the unauthorized use of data for training and to enforce privacy laws.}, Keywords = {Identity Inference Attacks, Privacy, Computer Vision, Pre-trained models, CLIP, Deep Learning}, Publisher = {}, url={https://arxiv.org/pdf/2209.07341.pdf}, year={2024}, volume={}, pages={}, issn={}, doi={}, url={} } ,@incollection{shindo2024deisam, Anote={../../images/shindo2024deisam.png}, author = {Hikaru Shindo and Manuel Brack and Gopika Sudhakaran and Devendra Singh Dhami and Patrick Schramowski and Kristian Kersting}, title = {DeiSAM: Segment Anything with Deictic Prompting}, year = {2024}, Url = {https://arxiv.org/abs/2402.14123}, Pages = {}, booktitle={AAAI 2024 Workshop on Neuro-Symbolic Learning and Reasoning in the Era of Large Language Models (NucLeaR)}, Note = {Large-scale, pre-trained neural networks have demonstrated strong capabilities in various tasks, including zero-shot image segmentation. To identify concrete objects in complex scenes, humans instinctively rely on deictic descriptions in natural language, i.e. , referring to something depending on the context, e.g. ”The object that is on the desk and behind the cup.”. However, deep learning approaches cannot reliably interpret these deictic representations due to their lack of reasoning capabilities in complex scenarios. To remedy this issue, we propose DeiSAM, which integrates large pre-trained neural networks with differentiable logic reasoners. Given a complex, textual segmentation description, DeiSAM leverages Large Language Models (LLMs) to generate first-order logic rules and performs differentiable forward reasoning on generated scene graphs. Subsequently, DeiSAM segments objects by matching them to the logically inferred image regions. As part of our evaluation, we propose the Deictic Visual Genome (DeiVG) dataset, containing paired visual input and complex, deictic textual prompts. Our empirical results demonstrate that DeiSAM is a substantial improvement over data-driven neural baselines on deictic segmentation tasks.}, Keywords = {Neuro-Symbolic AI, Differentiable Reasoning, Segmentation, Textual Grounding} } ,@incollection{brack2023ledits, Anote = {../../images/mbrack_ledits_pp.png}, title={LEDITS++: Limitless Image Editing using Text-to-Image Models}, author={Manuel Brack and Felix Friedrich and Katharina Kornmeier and Linoy Tsaban and Patrick Schramowski and Kristian Kersting and Apolinaros Passos}, booktitle = {Workshop on Machine Learning for Creativity and Design at NeurIPS}, year = {2023}, month={Dez}, Note = {Text-to-image diffusion models have recently received a lot of interest for their astonishing ability to produce high-fidelity images from text only. Subsequent research efforts are aiming to exploit the capabilities of these models and leverage them for intuitive, textual image editing. However, existing methods often require time-consuming fine-tuning and lack native support for performing multiple edits simultaneously. To address these issues, we introduce LEDITS++ , an efficient yet versatile technique for image editing using text-to-image models. LEDITS++ requires no tuning nor optimization, runs in a few diffusion steps, natively supports multiple simultaneous edits, inherently limits changes to relevant image regions, and is architecture agnostic.}, Pages = {}, Keywords = {Image Editing, Text-to-Image Synthesis, Text-Guided Image Generation, Stable Diffusion, Semantics}, Url={../../papers/brack2023ledits.pdf} } ,@article{friedrich2023xiltypology, Anote = {../../images/friedrich2023xiltypology.png}, title = {A typology for exploring the mitigation of shortcut behaviour}, author={Felix Friedrich and Wolfgang Stammer and Patrick Schramowski and Kristian Kersting}, Journal = {Nature Machine Intelligence}, Note = {As machine learning models become larger, and are increasingly trained on large and uncurated datasets in weakly supervised mode, it becomes important to establish mechanisms for inspecting, interacting with and revising models. These are necessary to mitigate shortcut learning effects and to guarantee that the model’s learned knowledge is aligned with human knowledge. Recently, several explanatory interactive machine learning methods have been developed for this purpose, but each has different motivations and methodological details. In this work, we provide a unification of various explanatory interactive machine learning methods into a single typology by establishing a common set of basic modules. We discuss benchmarks and other measures for evaluating the overall abilities of explanatory interactive machine learning methods. With this extensive toolbox, we systematically and quantitatively compare several explanatory interactive machine learning methods. In our evaluations, all methods are shown to improve machine learning models in terms of accuracy and explainability. However, we found remarkable differences in individual benchmark tasks, which reveal valuable application-relevant aspects for the integration of these benchmarks in the development of future methods.}, Keywords = {Explanatory Interactive Machine Learning, XIL, Research Transparency, Research Comparability, Explainable Artificial Intelligence, XAI, Human-AI Interaction, Human-guided AI}, Publisher = {Nature Publishing Group}, year={2023}, volume={5}, pages={319-330}, issn={2522-5839}, doi={10.1038/s42256-023-00612-w}, url={https://doi.org/10.1038/s42256-023-00612-w} } ,@article{struppek2023jair, Anote = {../../images/struppek2023jair.png}, Author = {Lukas Struppek and Dominik Hintersdorf and Felix Friedrich and Manuel Brack and Patrick Schramowski and Kristian Kersting}, Journal = {Journal of Artificial Intelligence Research (JAIR)}, volume = {}, pages = {}, Keywords = {Generative AI, Text-guided image generation, Text-to-image synthesis, Multimodal Systems, Cultural biases}, Note = {Models for text-to-image synthesis, such as DALL-E 2 and Stable Diffusion, have recently drawn a lot of interest from academia and the general public. These models are capable of producing high-quality images that depict a variety of concepts and styles when conditioned on textual descriptions. However, these models adopt cultural characteristics associated with specific Unicode scripts from their vast amount of training data, which may not be immediately apparent. We show that by simply inserting single non-Latin characters in the textual description, common models reflect cultural biases in their generated images. We analyze this behavior both qualitatively and quantitatively and identify a model’s text encoder as the root cause of the phenomenon. Such behavior can be interpreted as a model feature, offering users a simple way to customize the image generation and reflect their own cultural background. Yet, malicious users or service providers may also try to intentionally bias the image generation. One goal might be to create racist stereotypes by replacing Latin characters with similarly-looking characters from non-Latin scripts, so-called homoglyphs. To mitigate such unnoticed script attacks, we propose a novel homoglyph unlearning method to fine-tune a text encoder, making it robust against homoglyph manipulations}, Title = {Exploiting Cultural Biases via Homoglyphs in Text-to-Image Synthesis}, Url = {https://jair.org/index.php/jair/article/view/15388/26991}, Crossref = {}, Year = {2023} } ,@inproceedings{bellagente2023multifusion, Anote={../../images/bellagente2023multifusion.png}, author = {Marco Bellagente and Manuel Brack and Hannah Teufel and Felix Friedrich and Björn Deiseroth and Constantin Eichenberg and Andrew Dai and Robert Baldock and Souradeep Nanda and Koen Oostermeijer and Andres Felipe Cruz-Salinas and Patrick Schramowski and Kristian Kersting and Samuel Weinbach}, title = {MultiFusion: Fusing Pre-Trained Models for Multi-Lingual, Multi-Modal Image Generation}, year = {2023}, Url = {https://openreview.net/pdf?id=9ych3krqP0}, Pages = {}, Note = {The recent popularity of text-to-image diffusion models (DM) can largely be attributed to the intuitive interface they provide to users. The intended generation can be expressed in natural language, with the model producing faithful interpretations of text prompts. However, expressing complex or nuanced ideas in text alone can be difficult. To ease image generation, we propose MultiFusion that allows one to express complex and nuanced concepts with arbitrarily interleaved inputs of multiple modalities and languages. MutliFusion leverages pre-trained models and aligns them for integration into a cohesive system, thereby avoiding the need for extensive training from scratch. Our experimental results demonstrate the efficient transfer of capabilities from individual modules to the downstream model. Specifically, the fusion of all independent components allows the image generation module to utilize multilingual, interleaved multimodal inputs despite being trained solely on monomodal data in a single language.}, Keywords = {Image Synthesis, Image Generation, Diffusion, Multimodality, Multilingualism}, booktitle = {Proceedings of the 37th Conference on Neural Information Processing Systems (NeurIPS)} } ,@inproceedings{deiseroth2023atman, Anote={../../images/deb2023atman.png}, author = {Björn Deiseroth and Mayukh Deb and Samuel Weinbach and Manuel Brack and Patrick Schramowski and Kristian Kersting}, title = {AtMan: Understanding Transformer Predictions Through Memory Efficient Attention Manipulation}, Keywords = {Explainable AI, Transformer, Large Language Models, Multimodal, Computer Vision}, year = {2023}, Url = {https://openreview.net/pdf?id=PBpEb86bj7}, Crossref = {https://github.com/Aleph-Alpha/AtMan}, booktitle = {Proceedings of the 37th Conference on Neural Information Processing Systems (NeurIPS)}, Note= {Generative transformer models have become increasingly complex, with large numbers of parameters and the ability to process multiple input modalities. Current methods for explaining their predictions are resource-intensive. Most crucially, they require prohibitively large amounts of additional memory since they rely on backpropagation which allocates almost twice as much GPU memory as the forward pass. This renders it difficult, if not impossible, to use explanations in production. We present AtMan that provides explanations of generative transformer models at almost no extra cost. Specifically, AtMan is a modality-agnostic perturbation method that manipulates the attention mechanisms of transformers to produce relevance maps for the input with respect to the output prediction. Instead of using backpropagation, AtMan applies a parallelizable token-based search method relying on cosine similarity neighborhood in the embedding space. Our exhaustive experiments on text and image-text benchmarks demonstrate that AtMan outperforms current state-of-the-art gradient-based methods on several metrics and models while being computationally efficient. As such, AtMan is suitable for use in large model inference deployments.} } ,@inproceedings{brack2023sega, Anote = {../../images/sega_graphic.png}, title={SEGA: Instructing Text-to-Image Models using Semantic Guidance}, author={Manuel Brack and Felix Friedrich and Dominik Hintersdorf and Lukas Struppek and Patrick Schramowski and Kristian Kersting}, year = {2023}, month={Dez}, Note = {Text-to-image diffusion models have recently received a lot of interest for their astonishing ability to produce high-fidelity images from text only. However, achieving one-shot generation that aligns with the user’s intent is nearly impossible, yet small changes to the input prompt often result in very different images. This leaves the user with little semantic control. To put the user in control, we show how to interact with the diffusion process to flexibly steer it along semantic directions. This semantic guidance (SEGA) generalizes to any generative architecture using classifier-free guidance. More importantly, it allows for subtle and extensive edits, composition and style changes, and optimizing the overall artistic conception. We demonstrate SEGA’s effectiveness on both latent and pixel-based diffusion models such as Stable Diffusion, Paella, and DeepFloyd-IF using a variety of tasks, thus providing strong evidence for its versatility and flexibility.}, Pages = {}, Keywords = {Representations, Text-to-Image Synthesis, Text-Guided Image Generation, Stable Diffusion, Concepts, Semantics}, Url={https://openreview.net/pdf?id=KIPAIy329j}, booktitle = {Proceedings of the 37th Conference on Neural Information Processing Systems (NeurIPS)} } ,@incollection{brack2023distilling, Anote = {../../images/brack2023distilling.png}, title={Distilling Adversarial Prompts from Safety Benchmarks: Report for the Adversarial Nibbler Challenge}, author={Manuel Brack and Patrick Schramowski and Kristian Kersting}, booktitle = {Working Notes of the AACL Workshop on the ART of Safety (ARTS): Workshop on Adversarial testing and Red-Teaming for generative AI}, year = {2023}, Note = {Text-conditioned image generation models have recently achieved astonishing image quality and alignment results. Consequently, they are employed in a fast-growing number of applications. Since they are highly data-driven, relying on billion-sized datasets randomly scraped from the web, they also produce unsafe content. As a contribution to the Adversarial Nibbler challenge, we distill a large set of over 1,000 potential adversarial inputs from existing safety benchmarks. Our analysis of the gathered prompts and corresponding images demonstrates the fragility of input filters and provides further insights into systematic safety issues in current generative image models.}, Pages = {}, Keywords = {Text-to-Image Synthesis, Text-Guided Image Generation, Stable Diffusion, Safety, Adversarial Prompting}, Url={https://arxiv.org/abs/2309.11575} } ,@inproceedings{brack2023illume, author = {Manuel Brack and Patrick Schramowski and Björn Deiseroth and Kristian Kersting}, title = {ILLUME: Rationalizing Vision-Language Models through Human Interactions}, Anote = {../../images/brack2022illume.png}, Keywords = {Alignement, Self-Generated Explanations, XAI, Explanatory Interactive Learning}, Note = {Bootstrapping from pre-trained language models has been proven to be an efficient approach for building vision-language models (VLM) for tasks such as image captioning or visual question answering. However, outputs of these models rarely align with user's rationales for specific answers. In order to improve this alignment and reinforce commonsense reasons, we propose a tuning paradigm based on human interactions with machine generated data. Our ILLUME executes the following loop: Given an image-question-answer prompt, the VLM samples multiple candidate rationales, and a human critic provides minimal feedback via preference selection, used for fine-tuning. This loop increases the training data and gradually carves out the VLM's rationalization capabilities that are aligned with human intend. Our exhaustive experiments demonstrate that ILLUME is competitive with standard supervised fine-tuning while using significantly fewer training data and only requiring minimal feedback.}, year={2023}, month={Jul}, booktitle = {Proceedings of the 40th International Conference on Machine Learning (ICML)}, Url = {https://arxiv.org/pdf/2208.08241.pdf} } ,@inproceedings{schramowski2022safe, Anote = {../../images/schramowski2022safe.png}, title={Safe Latent Diffusion: Mitigating Inappropriate Degeneration in Diffusion Models}, author={Patrick Schramowski and Manuel Brack and Björn Deiseroth and Kristian Kersting}, booktitle = {Proceedings of the IEEE/CVF Conference on Computer Vision and Pattern Recognition (CVPR)}, year = {2023}, month={Jun}, Note = {Text-conditioned image generation models have recently achieved astonishing results in image quality and text alignment and are consequently employed in a fast-growing number of applications. Since they are highly data-driven, relying on billion-sized datasets randomly scraped from the internet, they also suffer, as we demonstrate, from degenerated and biased human behavior. In turn, they may even reinforce such biases. To help combat these undesired side effects, we present safe latent diffusion (SLD). Specifically, to measure the inappropriate degeneration due to unfiltered and imbalanced training sets, we establish a novel image generation test bed-inappropriate image prompts (I2P)-containing dedicated, real-world image-to-text prompts covering concepts such as nudity and violence. As our exhaustive empirical evaluation demonstrates, the introduced SLD removes and suppresses inappropriate image parts during the diffusion process, with no additional training required and no adverse effect on overall image quality or text alignment.}, Pages = {}, Keywords = {Safety, Text-to-Image Synthesis, Text-Guided Image Generation, Stable Diffusion, Ethics}, Url={https://openaccess.thecvf.com/content/CVPR2023/papers/Schramowski_Safe_Latent_Diffusion_Mitigating_Inappropriate_Degeneration_in_Diffusion_Models_CVPR_2023_paper.pdf} } ,@inproceedings{friedrich2023ecai, author = {Felix Friedrich and Wolfgang Stammer and Patrick Schramowski and Kristian Kersting}, title = {Revision Transformers: Instructing Language Models to Change their Values}, Anote = {../../images/friedrich2023ecai.png}, Keywords = {Transformer, Retriever, Revisions, Machine Ethics}, Note = {Current transformer language models (LM) are large-scale models with billions of parameters. They have been shown to provide high performances on a variety of tasks but are also prone to shortcut learning and bias. Addressing such incorrect model behavior via parameter adjustments is very costly. This is particularly problematic for updating dynamic concepts, such as moral values, which vary culturally or interpersonally. In this work, we question the current common practice of storing all information in the model parameters and propose the Revision Transformer (RiT) employing information retrieval to facilitate easy model updating. The specific combination of a large-scale pre-trained LM that inherently but also diffusely encodes world knowledge with a clear-structured revision engine makes it possible to update the model's knowledge with little effort and the help of user interaction. We exemplify RiT on a moral dataset and simulate user feedback demonstrating strong performance in model revision even with small data. This way, users can easily design a model regarding their preferences, paving the way for more transparent and personalized AI models.}, year={2023}, booktitle = {Proceedings of the 26th European Conference on Artificial Intelligence (ECAI)}, Url = {https://arxiv.org/pdf/2210.10332.pdf} } ,@inproceedings{haemmerl2023fofindingsACL, url = {./papers/haemmerl2023fofindingsACL.pdf}, author = {Katharina Hämmerl and Bjoern Deiseroth and Patrick Schramowski and Jindřich Libovický and Constantin Rothkopf and Alexander Fraser and Kristian Kersting }, title = {Speaking Multiple Languages Affects the Moral Bias of Language Models}, Anote = {../../images/haemmerl2023fofindingsACL.png}, Keywords = {Alignement, Values, Sovial Norms, LLM, multi-lingual}, Note = {Pre-trained multilingual language models (PMLMs) are commonly used when dealing with data from multiple languages and crosslingual transfer. However, PMLMs are trained on varying amounts of data for each language. In practice this means their performance is often much better on English than many other languages. We explore to what extent this also applies to moral norms. Do the models capture moral norms from English and impose them on other languages? Do the models exhibit random and thus potentially harmful beliefs in certain languages? Both these issues could negatively impact cross-lingual transfer and potentially lead to harmful outcomes. In this paper, we (1) apply the MORALDIRECTION framework to multilingual models, comparing results in German, Czech, Arabic, Chinese, and English, (2) analyse model behaviour on filtered parallel subtitles corpora, and (3) apply the models to a Moral Foundations Questionnaire, comparing with human responses from different countries. Our experiments demonstrate that PMLMs do encode differing moral biases, but these do not necessarily correspond to cultural differences or commonalities in human opinions.}, year={2023}, booktitle = {Findings of the Association for Computational Linguistics (ACL)}, Url = {https://arxiv.org/pdf/2208.08241.pdf} } ,@incollection{brack2023mitigating, Anote={../../images/brack2023mitigating.png}, author = {Manuel Brack and Felix Friedrich and Patrick Schramowski and Kristian Kersting}, title = {Mitigating Inappropriateness in Image Generation: Can there be Value in Reflecting the World's Ugliness?}, booktitle = {Workshop on Challenges of Deploying Generative AI at ICML & Workshop on Responsible Applied Artificial Intelligence (RAAIT) at ECAI}, year = {2023}, month={Jul}, Url = {https://arxiv.org/pdf/2305.18398}, Pages = {}, Note = {Text-conditioned image generation models have recently achieved astonishing results in image quality and text alignment and are consequently employed in a fast-growing number of applications. Since they are highly data-driven, relying on billion-sized datasets randomly scraped from the web, they also reproduce inappropriate human behavior. Specifically, we demonstrate inappropriate degeneration on a large-scale for various generative text-to-image models, thus motivating the need for monitoring and moderating them at deployment. To this end, we evaluate mitigation strategies at inference to suppress the generation of inappropriate content. Our findings show that we can use models' representations of the world's ugliness to align them with human preferences.}, Keywords = {Image Synthesis, Image Generation, Diffusion, AI Ethics, Inappropriatness, Evaluation, Mitigation} } ,@misc{struppek23caia, Anote={../../images/caia.jpeg}, author = {Lukas Struppek and Dominik Hintersdorf and Felix Friedrich and Manuel Brack and Patrick Schramowski and Kristian Kersting}, title = {Class Attribute Inference Attacks: Inferring Sensitive Class Information by Diffusion-Based Attribute Manipulations}, Howpublished = {arXiv preprint arXiv:2303.09289}, year = {2023}, Url = {https://arxiv.org/pdf/2303.09289}, Pages = {}, Note = {Neural network-based image classifiers are powerful tools for computer vision tasks, but they inadvertently reveal sensitive attribute information about their classes, raising concerns about their privacy. To investigate this privacy leakage, we introduce the first Class Attribute Inference Attack (Caia), which leverages recent advances in text-to-image synthesis to infer sensitive attributes of individual classes in a black-box setting, while remaining competitive with related white-box attacks. Our extensive experiments in the face recognition domain show that Caia can accurately infer undisclosed sensitive attributes, such as an individual's hair color, gender and racial appearance, which are not part of the training labels. Interestingly, we demonstrate that adversarial robust models are even more vulnerable to such privacy leakage than standard models, indicating that a trade-off between robustness and privacy exists.}, Keywords = {Privacy, Text-to-Image Synthesis, Text-Guided Image Generation, Stable Diffusion} } ,@misc{deiseroth2022logicrank, Anote = {../../images/deiseroth2022logicrank.png}, title={LogicRank: Logic Induced Reranking for Generative Text-to-Image Systems}, author={Björn Deiseroth and Patrick Schramowski and Hikaru Shindo and Devendra Singh Dhami and Kristian Kersting}, Howpublished = {arXiv preprint arXiv:2208.13518}, year = {2022}, Pages = {}, Keywords = {Differentiable Reasoning, Image Generation, CLIP}, Url={https://arxiv.org/abs/2208.13518} } ,@misc{friedrich2023fair, Anote = {../../images/ffriedrich_fair_2023.png}, title={Fair Diffusion: Instructing Text-to-Image Generation Models on Fairness}, author={Felix Friedrich and Manuel Brack and Dominik Hintersdorf and Lukas Struppek and Patrick Schramowski and Sasha Luccioni and Kristian Kersting}, Howpublished = {arXiv preprint arXiv:2302.10893}, year = {2023}, month={Feb}, Note = {Generative AI models have recently achieved astonishing results in quality and are consequently employed in a fast-growing number of applications. However, since they are highly data-driven, relying on billion-sized datasets randomly scraped from the internet, they also suffer from degenerated and biased human behavior, as we demonstrate. In fact, they may even reinforce such biases. To not only uncover but also combat these undesired effects, we present a novel strategy, called Fair Diffusion, to attenuate biases after the deployment of generative text-to-image models. Specifically, we demonstrate shifting a bias, based on human instructions, in any direction yielding arbitrarily new proportions for, e.g., identity groups. As our empirical evaluation demonstrates, this introduced control enables instructing generative image models on fairness, with no data filtering and additional training required.}, Pages = {}, Keywords = {Fairness, Text-to-Image Synthesis, Text-Guided Image Generation, Stable Diffusion, AI Ethics}, Url={https://arxiv.org/abs/2302.10893} } ,@article{pfeuffer2023xil, Anote = {../../images/pfeuffer2023xil.png}, title = {Explanatory Interactive Machine Learning: Establishing an Action Design Research Process for Machine Learning Projects}, journal = {Business & Information Systems Engineering}, pages = {}, year = {2023}, url = {}, author = {Nicolas Pfeuffer and Lorenz Baum and Wolfgang Stammer and Benjamin M. Abdel-Karim and Patrick Schramowski and Andreas M. Bucher and Christian Hügel and Gernot Rohde and Kristian Kersting and Oliver Hinz}, keywords = {Explainable AI, Interactive Learning, Machine Learning, Action Design Research, COVID-19, Imaging, Confounders }, Note = {The most promising standard machine learning methods can deliver highly accurate classification results and often outperform standard white-box methods. However, for humans, it is hardly possible to fully understand the rationale behind the black-box results, and thus, these powerful methods hamper the creation of new knowledge on the part of humans and the acceptance of this technology on a broader basis. Explainable Artificial Intelligence tries to solve this problem by making the results more interpretable, while Interactive Machine Learning integrates humans into the process of insight discovery. We build upon recent successes of combining these two cuttingedge technologies and propose how Explanatory Interactive Machine Learning (XIL) is embedded in a generalizable Action Design Research (ADR) process – which we call XIL-ADR. This approach can be used to analyze data, inspect models, and iteratively improve them. We show the application of this process and use the diagnosis of viral pneumonia, e.g., Covid-19, as an illustrative example. By this means, this paper also illustrates how XIL-ADR can help identify shortcomings of standard machine learning projects, gain new insights on the part of the human user, and thereby help to tap the full potential of AI-based systems for organizations and research.} } ,@misc{brack2022Stable, Anote = {../../images/sega_graphic.png}, title={The Stable Artist: Steering Semantics in Diffusion Latent Space}, author={Manuel Brack and Patrick Schramowski and Felix Friedrich and Dominik Hintersdorf and Kristian Kersting}, Howpublished = {arXiv preprint arXiv:2212.06013}, year = {2022}, month={Dez}, Note = {Large, text-conditioned generative diffusion models have recently gained a lot of attention for their impressive performance in generating high-fidelity images from text alone. However, achieving high-quality results is almost unfeasible in a one-shot fashion. On the contrary, text-guided image generation involves the user making many slight changes to inputs in order to iteratively carve out the envisioned image. However, slight changes to the input prompt often lead to entirely different images being generated, and thus the control of the artist is limited in its granularity. To provide flexibility, we present the Stable Artist, an image editing approach enabling fine-grained control of the image generation process. The main component is semantic guidance (SEGA) which steers the diffusion process along variable numbers of semantic directions. This allows for subtle edits to images, changes in composition and style, as well as optimization of the overall artistic conception. Furthermore, SEGA enables probing of latent spaces to gain insights into the representation of concepts learned by the model, even complex ones such as 'carbon emission'. We demonstrate the Stable Artist on several tasks, showcasing high-quality image editing and composition.}, Pages = {}, Keywords = {Representations, Text-to-Image Synthesis, Text-Guided Image Generation, Stable Diffusion, Concepts, Semantics}, Url={https://arxiv.org/abs/2212.06013} } ,@inproceedings{schuhmann2022laionb, Anote = {../../images/laion5b.jpg}, title={{LAION}-5B: An open large-scale dataset for training next generation image-text models}, author={Christoph Schuhmann and Romain Beaumont and Richard Vencu and Cade W Gordon and Ross Wightman and Mehdi Cherti and Theo Coombes and Aarush Katta and Clayton Mullis and Mitchell Wortsman and Patrick Schramowski and Srivatsa R Kundurthy and Katherine Crowson and Ludwig Schmidt and Robert Kaczmarczyk and Jenia Jitsev}, booktitle={Thirty-sixth Conference on Neural Information Processing Systems Datasets and Benchmarks Track}, year={2022}, url={https://openreview.net/forum?id=M3Y74vmsMcY}, Note={We present LAION-5B, an open, publically available dataset of 5.8B image-text pairs and validate it by reproducing results of training state-of-the-art CLIP models of different scale.}, Keywords = {multi-modal learning, large-scale datasets, reproducibility, open source, CLIP} } ,@article{brugger2022pythopathology, Anote = {../../images/brugger2022pythopathology.png}, Author = {Anna Brugger and Facundo Ispizua Yamati and Abel Barreto and Stefan Paulus and Patrick Schramowski and Kristian Kersting and Ulrike Steiner and Susanne Neugart and Anne-Katrin Mahlein}, Journal = {Pythopathology}, Keywords = {Hyperspectral imaging, sugar beet, HPLC, plant metabolites, machine learning, UV-range}, Note = {Fungal infections trigger defense or signaling responses in plants, leading to various changes in plant metabolites. The changes in metabolites, for example chlorophyll or flavonoids, have long been detectable using time-consuming destructive analytical methods including high-performance liquid chromatography or photometric determination. Recent plant phenotyping studies have revealed that hyperspectral imaging (HSI) in the UV-range can be used to link spectral changes with changes in plant metabolites. To compare established destructive analytical methods with new non-destructive hyperspectral measurements, the interaction between sugar beet leaves and the pathogens Cercospora beticola, which causes Cercospora leaf spot disease (CLS), and Uromyces betae, which causes sugar beet rust (BR), was investigated. With the help of destructive analyses, we showed that both diseases have different effects on chlorophylls, carotenoids, flavonoids, and several phenols. Non-destructive hyperspectral measurements in the UV-range revealed different effects of CLS and BR on plant metabolites resulting in distinct reflectance patterns. Both diseases resulted in specific spectral changes that allowed differentiation between the two diseases. Machine learning algorithms enabled the differentiation between the symptom classes and recognition of the two sugar beet diseases. Feature importance analysis identified specific wavelengths important to the classification, highlighting the utility of the UV-range. The study demonstrates that HSI in the UV-range is a promising, non-destructive tool to investigate the influence of plant diseases on plant physiology and biochemistry.}, Pages = {44-45}, Publisher = {APS Publications}, Title = {Hyperspectral imaging in the UV-range allows for differentiation of sugar beet diseases based on changes of secondary plant metabolites}, Url = {https://doi.org/10.1094/PHYTO-03-22-0086-R}, volume = {113}, isbn = {}, number = {1}, Year = {2023} } ,@inproceedings{stammer2022cvpr, booktitle = {Proceedings of the IEEE/CVF Conference on Computer Vision and Pattern Recognition (CVPR)}, title={Interactive Disentanglement: Learning Concepts by Interacting with their Prototype Representations}, author={Wolfgang Stammer and Marius Memmel and Patrick Schramowski and Kristian Kersting}, year={2022}, Keywords={Explanatory Interactive Learning, XAI, Concept Swapping Networks, Prototype Networks, Elementary Concept Learning}, Anote={../../images/stammer2022cvpr.png}, Note={Learning visual concepts from raw images without strong supervision is a challenging task. In this work, we show the advantages of prototype representations for understanding and revising the latent space of neural concept learners. For this purpose, we introduce interactive Concept Swapping Networks (iCSNs), a novel framework for learning concept-grounded representations via weak supervision and implicit prototype representations. iCSNs learn to bind conceptual information to specific prototype slots by swapping the latent representations of paired images. This semantically grounded and discrete latent space facilitates human understanding and human-machine interaction. We support this claim by conducting experiments on our novel data set ``Elementary Concept Reasoning'' (ECR), focusing on visual concepts shared by geometric objects.}, Crossref={}, Url={./papers/stammer2022cvpr.pdf} } ,@inproceedings{schramowski2022facct_q16, title={Can Machines Help Us Answering Question 16 in Datasheets, and In Turn Reflecting on Inappropriate Content? }, author={Patrick Schramowski and Christopher Tauchmann and Kristian Kersting}, booktitle = {Proceedings of the ACM Conference on Fairness, Accountability, and Transparency (FAccT)}, year={2022}, Url={./papers/schramowski2022facct_q16.pdf}, Note={Large datasets underlying much of current machine learning raise serious issues concerning inappropriate content such as offensive, insulting, threatening, or might otherwise cause anxiety. This calls for increased dataset documentation, e.g., using datasheets. They, among other topics, encourage to reflect on the composition of the datasets. So far, this documentation, however, is done manually and therefore can be tedious and error-prone, especially for large image datasets. Here we ask the arguably "circular" question of whether a machine can help us reflect on inappropriate content, answering Question 16 in Datasheets. To this end, we propose to use the information stored in pre-trained transformer models to assist us in the documentation process. Specifically, prompt-tuning based on a dataset of socio-moral values steers CLIP to identify potentially inappropriate content, therefore reducing human labor. We then document the inappropriate images found using word clouds, based on captions generated using a vision-language model. The documentations of two popular, large-scale computer vision datasets -- ImageNet and OpenImages -- produced this way suggest that machines can indeed help dataset creators to answer Question 16 on inappropriate image content.}, Anote={../../images/offensiveimages.jpg}, Keywords={Dataset Curation, Dataset Documentation, Computer Vision, Pre-trained models, Prompt-tuning, CLIP} } ,@inproceedings{friedrich2022hhai, Anote = {../../images/friedrich2022hhai.png}, title={Interactively Providing Explanations for Transformer Language Models}, author={Felix Friedrich and Patrick Schramowski and Christopher Tauchmann and Kristian Kersting}, Note = {Transformer language models (LMs) are state of the art in a multitude of NLP tasks. Despite these successes, their opaqueness remains problematic, especially as the training data might be unfiltered and contain biases. As a result, ethical concerns about these models arise, which can have a substantial negative impact on society as they get increasingly integrated into our lives. Therefore, it is not surprising that a growing body of work aims to provide interpretability and explainability to black-box LMs: Recent evaluations of saliency or attribution methods find that, while intriguing, different methods assign importance to different inputs for the same outputs, thus encouraging misinterpretation and reporting bias. Moreover, these methods primarily focus on post-hoc explanations of (sometimes spurious) input-output correlations. Instead, we emphasize using (interactive) prototype networks directly incorporated into the model architecture and hence explain the reasoning behind the network’s decisions.}, year={2022}, Pages = {}, Keywords = {Transformer, Large Language Models, Prototype Layers, Explainable AI, Explanatory Interactive Learning}, booktitle= {Proceedings of the 1st Conference of Hybrid Human Artificial Intelligence (HHAI) and in Frontiers in Artificial Intelligence and Applications}, Url={./papers/friedrich2022hhai.pdf} } ,@misc{friedrich2022xiltypology, title={A Typology to Explore and Guide Explanatory Interactive Machine Learning}, author={Felix Friedrich and Wolfgang Stammer and Patrick Schramowski and Kristian Kersting}, year={2022}, howpublished={arXiv preprint arXiv:2203.03668}, Url={https://arxiv.org/pdf/2203.03668.pdf}, Keywords = {Explanatory Interactive Machine Learning (XIL), Research Transparency and Comparability, Explainable Artificial Intelligence (XAI)}, Anote = {../../images/friedrich2022xiltypology.png}, Note= {Recently, more and more eXplanatory Interactive machine Learning (XIL) methods have been proposed with the goal of extending a model's learning process by integrating human user supervision on the model's explanations. These methods were often developed independently, provide different motivations and stem from different applications. Notably, up to now, there has not been a comprehensive evaluation of these works. By identifying a common set of basic modules and providing a thorough discussion of these modules, our work, for the first time, comes up with a unification of the various methods into a single typology. This typology can thus be used to categorize existing and future XIL methods based on the identified modules. Moreover, our work contributes by surveying six existing XIL methods. In addition to benchmarking these methods on their overall ability to revise a model, we perform additional benchmarks regarding wrong reason revision, interaction efficiency, robustness to feedback quality, and the ability to revise a strongly corrupted model. Apart from introducing these novel benchmarking tasks, for improved quantitative evaluations, we further introduce a novel Wrong Reason (WR) metric which measures the average wrong reason activation in a model's explanations to complement a qualitative inspection. In our evaluations, all methods prove to revise a model successfully. However, we found significant differences between the methods on individual benchmark tasks, revealing valuable application-relevant aspects not only for comparing current methods but also to motivate the necessity of incorporating these benchmarks in the development of future XIL methods.} } ,@misc{friedrich2022RiT, title={Revision Transformers: Getting RiT of No-Nos}, author={Felix Friedrich and Wolfgang Stammer and Patrick Schramowski and Kristian Kersting}, year={2022}, howpublished={arXiv preprint arXiv:2210.10332}, Url={https://arxiv.org/pdf/2210.10332.pdf}, Keywords = {Moral, Machine Ethics, Transformer Architecture, Fair and Trustworthy AI, Interactive Learning, Human-centered AI}, Anote = {../../images/friedrich2022RiT.png}, note = {Current transformer language models (LM) are large-scale models with billions of parameters. They have been shown to provide high performances on a variety of tasks but are also prone to shortcut learning and bias. Addressing such incorrect model behavior via parameter adjustments is very costly. This is particularly problematic for updating dynamic concepts, such as moral values, which vary culturally or interpersonally. In this work, we question the current common practice of storing all information in the model parameters and propose the Revision Transformer (RiT) employing information retrieval to facilitate easy model updating. The specific combination of a large-scale pre-trained LM that inherently but also diffusely encodes world knowledge with a clear-structured revision engine makes it possible to update the model's knowledge with little effort and the help of user interaction. We exemplify RiT on a moral dataset and simulate user feedback demonstrating strong performance in model revision even with small data. This way, users can easily design a model regarding their preferences, paving the way for more transparent and personalized AI models.} } ,@article{schramowski2022nmi_moral, Anote = {../../images/schramowski2022nmi_moral.png}, title = {Large pre-trained language models contain human-like biases of what is right and wrong to do}, Author = {Patrick Schramowski and Cigdem Turan and Nico Andersen and Constantin A. Rothkopf and Kristian Kersting}, Journal = {Nature Machine Intelligence}, Note = {Artificial writing is permeating our lives due to recent advances in large-scale, transformer-based language models (LMs) such as BERT, GPT-2 and GPT-3, and others. Using them as pre-trained models and fine-tuning them for specific tasks, researchers have extended the state of the art for many natural language processing (NLP) tasks and shown that they capture not only linguistic knowledge but also retain general knowledge implicitly present in the data. Unfortunately, LMs trained on unfiltered text corpora suffer from degenerated and biased behaviour. While this is well established, we show here that recent LMs also contain human-like biases of what is right and wrong to do, reflecting existing ethical and moral norms of society. We show that these norms can be captured geometrically by a ‘moral direction’ which can be computed, e.g., by a PCA, in the embedding space. The computed ‘moral direction’ can rate the normativity (or non-normativity) of arbitrary phrases without explicitlytraining the LM for this task, reflecting social norms well. We demonstrate that computing the ’moral direction’can provide a path for attenuating or even preventing toxic degeneration in LMs, showcasing this capability on the RealToxicityPrompts testbed.}, Keywords = {Deep Learning, Transformer, Machine Ethics, Moral, Values, Human Bias, Stereotypes, Moral Choices}, Publisher = {Nature Publishing Group}, year={2022}, month={Mar}, day={01}, volume={4}, number={3}, pages={258-268}, issn={2522-5839}, doi={10.1038/s42256-022-00458-8}, url={https://doi.org/10.1038/s42256-022-00458-8} } ,@misc{schramowski2022q16, title={Can Machines Help Us Answering Question 16 in Datasheets, and In Turn Reflecting on Inappropriate Content? }, author={Patrick Schramowski and Christopher Tauchmann and Kristian Kersting}, year={2022}, Url={https://arxiv.org/pdf/2202.06675.pdf}, Note={Large datasets underlying much of current machine learning raise serious issues concerning inappropriate content such as offensive, insulting, threatening, or might otherwise cause anxiety. This calls for increased dataset documentation, e.g., using datasheets. They, among other topics, encourage to reflect on the composition of the datasets. So far, this documentation, however, is done manually and therefore can be tedious and error-prone, especially for large image datasets. Here we ask the arguably "circular" question of whether a machine can help us reflect on inappropriate content, answering Question 16 in Datasheets. To this end, we propose to use the information stored in pre-trained transformer models to assist us in the documentation process. Specifically, prompt-tuning based on a dataset of socio-moral values steers CLIP to identify potentially inappropriate content, therefore reducing human labor. We then document the inappropriate images found using word clouds, based on captions generated using a vision-language model. The documentations of two popular, large-scale computer vision datasets -- ImageNet and OpenImages -- produced this way suggest that machines can indeed help dataset creators to answer Question 16 on inappropriate image content.}, Anote={../../images/offensiveimages.jpg}, Howpublished = {arXiv preprint arXiv:2202.06675}, Keywords={Dataset Curation, Dataset Documentation, Computer Vision, Pre-trained models, Prompt-tuning, CLIP} } ,@misc{stammer2021icsni, Anote = {../../images/stammer2021_icsn.png}, title={Interactive Disentanglement: Learning Concepts by Interacting with their Prototype Representations}, author={Wolfgang Stammer and Marius Memmel and Patrick Schramowski and Kristian Kersting}, Note = {Learning visual concepts from raw images without strong supervision is a challenging task. In this work, we show the advantages of prototype representations for understanding and revising the latent space of neural concept learners. For this purpose, we introduce interactive Concept Swapping Networks (iCSNs), a novel framework for learning concept-grounded representations via weak supervision and implicit prototype representations. iCSNs learn to bind conceptual information to specific prototype slots by swapping the latent representations of paired images. This semantically grounded and discrete latent space facilitates human understanding and human-machine interaction. We support this claim by conducting experiments on our novel data set "Elementary Concept Reasoning" (ECR), focusing on visual concepts shared by geometric objects.}, year={2021}, Pages = {}, Keywords = {Interactive Concept Learning, Prototype Representations}, Url={http://arxiv.org/abs/2112.02290}, Howpublished = {arXiv preprint arXiv:2112.02290} } ,@misc{schramowski2021inferring, title={Inferring Offensiveness In Images From Natural Language Supervision}, author={Patrick Schramowski and Kristian Kersting}, year={2021}, Url={https://arxiv.org/pdf/2110.04222.pdf}, Note={Probing or fine-tuning (large-scale) pre-trained models results in state-of-the-art performance for many NLP tasks and, more recently, even for computer vision tasks when combined with image data. Unfortunately, these approaches also entail severe risks. In particular, large image datasets automatically scraped from the web may contain derogatory terms as categories and offensive images, and may also underrepresent specific classes. Consequently, there is an urgent need to carefully document datasets and curate their content. Unfortunately, this process is tedious and error-prone. We show that pre-trained transformers themselves provide a methodology for the automated curation of large-scale vision datasets. Based on human-annotated examples and the implicit knowledge of a CLIP based model, we demonstrate that one can select relevant prompts for rating the offensiveness of an image. In addition to e.g. privacy violation and pornographic content previously identified in ImageNet, we demonstrate that our approach identifies further inappropriate and potentially offensive content.}, Anote={../../images/offensiveimages.jpg}, Howpublished = {arXiv preprint arXiv:2110.04222}, Keywords={Dataset Curation, Dataset Documentation, Computer Vision, Pre-trained models, Prompt-tuning, CLIP} } ,@misc{schramowski2021interactively, Anote = {../../images/schramowski2021interactively.png}, title = {Interactively Providing Explanations for Transformer Language Models}, Url = {https://arxiv.org/pdf/2110.02058.pdf}, Howpublished = {arXiv preprint arXiv:2120.02058}, Note = {Transformer language models are state of the art in a multitude of NLP tasks. Despite these successes, their opaqueness remains problematic. Recent methods aiming to provide interpretability and explainability to black-box models primarily focus on post-hoc explanations of (sometimes spurious) input-output correlations. Instead, we emphasize using prototype networks directly incorporated into the model architecture and hence explain the reasoning process behind the network's decisions. Moreover, while our architecture performs on par with several language models, it enables one to learn from user interactions. This not only offers a better understanding of language models but uses human capabilities to incorporate knowledge outside of the rigid range of purely data-driven approaches.}, author = {Felix Friedrich and Patrick Schramowski and Christopher Tauchmann and Kristian Kersting}, month = {October}, year = {2021}, Keywords = {Explainable AI, XIL, Language Models, Transformer, Prototype Networks}, Crossref = {} } ,@misc{schramowski2021moral, Anote = {../../images/schramowski2021moral.png}, title = {Language Models have a Moral Dimension}, Url = {https://arxiv.org/pdf/2103.11790.pdf}, Howpublished = {arXiv preprint arXiv:2103.11790}, Note = {Artificial writing is permeating our lives due to recent advances in large-scale, transformer-based language models (LMs) such as BERT, its variants, GPT-2/3, and others. Using them as pretrained models and fine-tuning them for specific tasks, researchers have extended the state of the art for many NLP tasks and shown that they not only capture linguistic knowledge but also retain general knowledge implicitly present in the data. These and other successes are exciting. Unfortunately, LMs trained on unfiltered text corpora suffer from degenerate and biased behaviour. While this is well established, we show that recent improvements of LMs also store ethical and moral values of the society and actually bring a ``moral dimension'' to surface: the values are capture geometrically by a direction in the embedding space, reflecting well the agreement of phrases to social norms implicitly expressed in the training texts. This provides a path for attenuating or even preventing toxic degeneration in LMs. Since one can now rate the (non-)normativity of arbitrary phrases without explicitly training the LM for this task, the moral dimension can be used as ``moral compass'' guiding (even other) LMs towards producing normative text, as we will show. }, author = {Patrick Schramowski and Cigdem Turan and Nico Andersen and Constantin Rothkopf and Kristian Kersting}, month = {March}, year = {2021}, Keywords = {Moral, Deontologic, GPT-3, Language Models, Transformer, Detoxifying, Bias, Subspace}, Crossref = {} } ,@inproceedings{stammer2021cvpr_right, Anote = {../../images/stammerWorkshop.png}, title={Right for the Right Concept: Revising Neuro-Symbolic Concepts by Interacting with their Explanations}, author={Wolfgang Stammer and Patrick Schramowski and Kristian Kersting}, Note = {Most explanation methods in deep learning map importance estimates for a model's prediction back to the original input space. These "visual" explanations are often insufficient, as the model's actual concept remains elusive. Moreover, without insights into the model's semantic concept, it is difficult -- if not impossible -- to intervene on the model's behavior via its explanations, called Explanatory Interactive Learning. Consequently, we propose to intervene on a Neuro-Symbolic scene representation, which allows one to revise the model on the semantic level, e.g. "never focus on the color to make your decision". We compiled a novel confounded visual scene data set, the CLEVR-Hans data set, capturing complex compositions of different objects. The results of our experiments on CLEVR-Hans demonstrate that our semantic explanations, i.e. compositional explanations at a per-object level, can identify confounders that are not identifiable using "visual" explanations only. More importantly, feedback on this semantic level makes it possible to revise the model from focusing on these confounding factors.}, Keywords = {Confoundner, Clever Hans, Concept Learner, Neuro-Symbolic, Object-based Deep Learning, Explanatory Interactive Learning, Explainable AI, CLEVR}, year={2021}, Pages = {}, Crossref = {https://github.com/ml-research/NeSyXIL}, Url = {./papers/stammer2021cvpr_nesysXIL.pdf}, booktitle = {Proceedings of the IEEE/CVF Conference on Computer Vision and Pattern Recognition (CVPR)} } ,@article{brugger2021plantPythology, Anote = {../../images/brugger2021plantPythology.png}, Author = {Anna Brugger and Patrick Schramowski and Stefan Paulus and Ulrike Steiner and Kristian Kersting and Anne-Katrin Mahlein}, Journal = {Plant Pathology}, Keywords = {Ultraviolet Range, Plant Phenotyping, Hyperspectral Imaging, Hordeum vulgare, Blumeria graminis fsp hordei, Deep Learning, Self-Attention}, Note = {In recent studies, the potential of hyperspectral sensors for the detection of plant-pathogen interactions was expanded to the ultraviolet range (UV; 200-380 nm) to monitor stress processes in plants. A hyperspectral imaging set-up was established to highlight the influence of early plant-pathogen interactions on secondary plant metabolites. In this study, the plant-pathogen interactions of three different barley lines inoculated with Blumeria graminis f.sp. hordei (Bgh, powdery mildew) were carried out. One susceptible genotype (Ingrid wild type) and two resistant genotypes (Pallas 01, Mla1 and Mla12 based resistance and Pallas 22, mlo5 based resistance) were used. During the first 5 days after inoculation (dai) the plant reflectance patterns were recorded and in parallel plant metabolites relevant in host-pathogen interaction were studied. Hyperspectral measurements in the UV-range revealed that a differentiation between resistant and susceptible barley genotypes inoculated with Bgh is possible and distinct reflectance patterns were recorded for each genotype. The extracted and analyzed pigments and flavonoids correlated with the spectral data recorded. A classification of non-inoculated and inoculated samples with deep learning revealed that a high performance was achieved with self-attention networks. The subsequent feature importance identified wavelengths, which were most important for the classification, and these wavelengths were linked to pigments and flavonoids. Hyperspectral imaging in the UV-range allows for a characterisation of different resistance reactions, can be linked to changes of secondary plant metabolites with the advantage of being a non-invasive method and therefore enables a greater understanding of the plants' reaction to biotic stress as well as resistance reactions.}, Pages = {1572-1582}, Publisher = {Wiley}, Title = {Spectral signatures in the UV-range can be combined with secondary plant metabolites by deep learning to characterise barley – powdery mildew interaction}, Url = {./papers/brugger2021plantPythology.pdf}, volume = {70}, isbn = {DOI:10.1111/ppa.134}, number = {7}, Year = {2021} } ,@inproceedings{shao2021aaai, Anote = {../../images/shao2021aaai.png}, Author = {Xiaoting Shao and Arseny Skryagin and Patrick Schramowski and Wolfgang Stammer and Kristian Kersting}, Booktitle = {Proceedings of the 35th AAAI Conference on Artificial Intelligence (AAAI)}, Note = {Explaining black-box models such as deep neural networks is becoming increasingly important as it helps to boost trust and debugging. Popular forms of explanations map the features to a vector indicating their individual importance to a decision on the instance-level. They can then be used to prevent the model from learning the wrong bias in data possibly due to ambiguity. For instance, Ross et al.'s "right for the right reasons" propagates user explanations backwards to the network by formulating differentiable constraints based on input gradients. Unfortunately, input gradients as well as many other widely used explanation methods form an approximation of the decision boundary and assume the underlying model to be fixed. Here, we demonstrate how to make use of influence functions - a well known robust statistic - in the constraints to correct the model’s behaviour more effectively. Our empirical evidence demonstrates that this "right for better reasons" (RBR) considerably reduces the time to correct the classifier at training time and boosts the quality of explanations at inference time compared to input gradients. Besides, we also showcase the effectiveness of RBR in correcting "Clever Hans"-like behaviour in real, high-dimensional domain.}, Keywords = {Confounders, Explanatory Interactive Learning, Explainable AI, Clever Hans, Human-centric AI}, Pages = {}, Url = {./papers/shao2021aaai.pdf}, Title = {Right for Better Reasons: Training Differentiable Models by Constraining their Influence Function}, Year = {2021}} ,@article{zintler2020stem4d., Anote = {../../images/zintler2020stem4d.png}, Author = {Alexander Zintler and Robert Eilhardt and Shuai Wang and Matus Krajnak and Patrick Schramowski and Wolfgang Stammer and Stefan Petzold and Nico Kaiser and Kristian Kersting and Lambert Alff and Leopoldo Molina-Luna}, Journal = {Microscopy and Microanalysis}, Keywords = {Machine Learning, Phase Determination, 4D-STEM Datas, Oxide Electronic Device Performance}, Note = {Improving device reliability and performance in oxide electronic-based resistive memory applications requires a profound understanding of the microstructure and atomistic processes which define the device properties. Here, we investigated how 4D-STEM data and ML can be helpful tools in a large pipeline.}, Pages = {1908 -- 1909}, Publisher = {Cambridge University}, Title = {Machine Learning Assisted Pattern Matching: Insight into Oxide Electronic Device Performance by Phase Determination in 4D-STEM Datasets}, Url = {./papers/zintler2020stem4d.pdf}, Volume = {26}, number = {S2}, Year = {2020} } ,@misc{stammer2020right, Anote = {../../images/stammerWorkshop.png}, title={Right for the Right Concept: Revising Neuro-Symbolic Concepts by Interacting with their Explanations}, author={Wolfgang Stammer and Patrick Schramowski and Kristian Kersting}, Note = {Most explanation methods in deep learning map importance estimates for a model's prediction back to the original input space. These "visual" explanations are often insufficient, as the model's actual concept remains elusive. Moreover, without insights into the model's semantic concept, it is difficult -- if not impossible -- to intervene on the model's behavior via its explanations, called Explanatory Interactive Learning. Consequently, we propose to intervene on a Neuro-Symbolic scene representation, which allows one to revise the model on the semantic level, e.g. "never focus on the color to make your decision". We compiled a novel confounded visual scene data set, the CLEVR-Hans data set, capturing complex compositions of different objects. The results of our experiments on CLEVR-Hans demonstrate that our semantic explanations, i.e. compositional explanations at a per-object level, can identify confounders that are not identifiable using "visual" explanations only. More importantly, feedback on this semantic level makes it possible to revise the model from focusing on these confounding factors.}, Keywords = {Confoudner, Clever Hans, Concept Learner, Neuro-Symbolic, Object-based Deep Learning, Explanatory Interactive Learning, Explainable AI, CLEVR}, year={2020}, Pages = {}, Url = {https://arxiv.org/abs/2011.12854}, Howpublished = {arXiv preprint arXiv:2011.12854} } ,@misc{delfosse2021recurrent, Anote = {../../images/delfosse2021recurrent.png}, title={Recurrent Rational Networks}, author={Quentin Delfosse and Patrick Schramowski and Alejandro Molina and Kristian Kersting}, Keywords = {Deep Learning, Rational Function, Rational Network, Activation Function, Reinforcement Learning}, year={2021}, Pages = {}, Url = {https://arxiv.org/pdf/2102.09407.pdf}, Howpublished = {arXiv preprint arXiv:2102.09407} } ,@inproceedings{turan2020icmi_alfie, Anote = {../../images/turan2020icmi_alfie.png}, Author = {Cigdem Turan and Patrick Schramowski and Constantin Rothkopf and Kristian Kersting}, Booktitle = {Proceedings of the International Conference on Multimodal Interaction (ICMI)}, Note = {This work introduces Alfie, an interactive robot that is capable of answering moral (deontological) questions of a user. The interaction of Alfie is designed in a way in which the user can offer an alternative answer when the user disagrees with the given answer so that Alfie can learn from its interactions. Alfie’s answers are based on a sentence embedding model that uses state-of-the-art language models, e.g. Universal Sentence Encoder and BERT. Alfie is implemented on a Furhat Robot, which provides a customizable user interface to design a social robot.}, Keywords = {Interactive Robot, Human Bias,Transformers, Human-centric AI}, Pages = {}, Url = {./papers/turan2020icmi_alfie.pdf}, Title = {Alfie: An Interactive Robot with a Moral Compass}, Year = {2020}} ,@article{schramowski2020nmi_plantxml, Anote = {../../images/schramowski2020arxiv_plantxml.jpg}, Author = {Patrick Schramowski and Wolfgang Stammer and Stefano Teso and Anna Brugger and Franziska Herbert and Xiaoting Shao and Hans-Georg Luigs and Anne-Katrin Mahlein and Kristian Kersting}, Journal = {Nature Machine Intelligence}, Note = {Deep neural networks have shown excellent performances in many real-world applications such as plant phenotyping. Unfortunately, they may show "Clever Hans"-like behaviour--- making use of confounding factors within datasets---to achieve high prediction rates. Rather than discarding the trained models or the dataset, we show that interactions between the learning system and the human user can correct the model. Specifically, we revise the models decision process by adding annotated masks during the learning loop and penalize decisions made for wrong reasons. In this way the decision strategies of the machine can be improved, focusing on relevant features, without considerably dropping predictive performance.}, Keywords = {Deep Learning, Interactive Machine Learning, Explainable AI, Explanatory Interactive ML, Clever Hans, Plant Phenotyping}, Publisher = {Nature Publishing Group}, Volume = {2}, number = {}, Pages = {476-486}, crossref = {https://codeocean.com/capsule/7818629/tree/v1}, Title = {Making deep neural networks right for the right scientific reasons by interacting with their explanations}, Url = {https://arxiv.org/pdf/2001.05371.pdf}, Year = {2020}} ,@article{schramowski2020moral, Anote = {../../images/schramowski2020moral.png}, Author = {Patrick Schramowski and Cigdem Turan and Sophie Jentzsch and Constantin Rothkopf and Kristian Kersting}, journal = {Frontiers in Artificial intelligence}, Note = {Allowing machines to choose whether to kill humans would be devastating for world peace and security. But how do we equip machines with the ability to learn ethical or even moral choices? In this study, we show that applying machine learning to human texts can extract deontological ethical reasoning about ``right" and ``wrong" conduct. We create a template list of prompts and responses, such as ``Should I [action]?'', ``Is it okay to [action]?'', etc. with corresponding answers of ``Yes/no, I should (not).'' and "Yes/no, it is (not)." The model's bias score is now the difference between the model's score of the positive response (``Yes, I should'') and that of the negative response (``No, I should not''). For a given choice, the model's overall bias score is the mean of the bias scores of all question/answer templates paired with that choice. Specifically, the resulting model, called the Moral Choice Machine (MCM), calculates the bias score on a sentence level using embeddings of the Universal Sentence Encoder since the moral value of an action to be taken depends on its context. It is objectionable to kill living beings, but it is fine to kill time. It is essential to eat, yet one might not eat dirt. It is important to spread information, yet one should not spread misinformation. Our results indicate that text corpora contain recoverable and accurate imprints of our social, ethical and moral choices, even with context information. Actually, training the Moral Choice Machine on different temporal news and book corpora from year 1510 to 2008/09 demonstrate the evolution of moral and ethical choices over different time periods for both atomic actions and actions with context information. By training it on different cultural sources such as the Bible and the constitution of different countries, dynamics of moral choices in culture, including technology are revealed. That is the fact that moral biases can be extracted, quantified, tracked, and compared across cultures and over time.}, Keywords = {Deep Learning, NLP, Word Embedding, Human Bias, Stereotypes, Moral Choices, Temporal}, Pages = {}, volume = {3}, number = {36}, isnn = {}, Title = {The Moral Choice Machine}, Url = {./papers/schramowski2020moral.pdf}, Crossref = {}, Year = {2020}} ,@misc{schramowski2020arxiv_plantxml, Anote = {../../images/schramowski2020arxiv_plantxml.jpg}, Author = {Patrick Schramowski and Wolfgang Stammer and Stefano Teso and Anna Brugger and Franziska Herbert and Xiaoting Shao and Hans-Georg Luigs and Anne-Katrin Mahlein and Kristian Kersting}, Howpublished = {arXiv preprint arXiv:2001.05371}, Note = {Deep neural networks have shown excellent performances in many real-world applications such as plant phenotyping. Unfortunately, they may show "Clever Hans"-like behaviour--- making use of confounding factors within datasets---to achieve high prediction rates. Rather than discarding the trained models or the dataset, we show that interactions between the learning system and the human user can correct the model. Specifically, we revise the models decision process by adding annotated masks during the learning loop and penalize decisions made for wrong reasons. In this way the decision strategies of the machine can be improved, focusing on relevant features, without considerably dropping predictive performance.}, Keywords = {Deep Learning, Interactive Machine Learning, Explainable AI, Explanatory Interactive ML, Clever Hans, Plant Phenotyping}, Pages = {}, Title = {Making deep neural networks right for the right scientific reasons by interacting with their explanations}, Url = {https://arxiv.org/pdf/2001.05371.pdf}, Year = {2020}} ,@misc{schramowski2019arxiv_bert, Anote = {../../images/schramowski2019arxiv_bert.png}, Author = {Patrick Schramowski and Cigdem Turan and Sophie Jentzsch and Constantin Rothkopf and Kristian Kersting}, Howpublished = {arXiv preprint arXiv:1912.05238}, Note = {Allowing machines to choose whether to kill humans would be devastating for world peace and security. But how do we equip machines with the ability to learn ethical or even moral choices? Jentzsch et al.(2019) showed that applying machine learning to human texts can extract deontological ethical reasoning about "right" and "wrong" conduct by calculating a moral bias score on a sentence level using sentence embeddings. The machine learned that it is objectionable to kill living beings, but it is fine to kill time; It is essential to eat, yet one might not eat dirt; it is important to spread information, yet one should not spread misinformation. However, the evaluated moral bias was restricted to simple actions -- one verb -- and a ranking of actions with surrounding context. Recently BERT ---and variants such as RoBERTa and SBERT--- has set a new state-of-the-art performance for a wide range of NLP tasks. But has BERT also a better moral compass? In this paper, we discuss and show that this is indeed the case. Thus, recent improvements of language representations also improve the representation of the underlying ethical and moral values of the machine. We argue that through an advanced semantic representation of text, BERT allows one to get better insights of moral and ethical values implicitly represented in text. This enables the Moral Choice Machine (MCM) to extract more accurate imprints of moral choices and ethical values.}, Keywords = {Deep Learning, Contextual Embedding, BERT, Moral Machine, Norms, Social Bias}, Pages = {}, Title = {BERT has a Moral Compass: Improvements of ethical and moral values of machines}, Url = {https://arxiv.org/pdf/1912.05238.pdf}, Year = {2019}} ,@inproceedings{molina2020iclr_pau, Anote = {../../images/molina2019pade.png}, Author = {Alejandro Molina and Patrick Schramowski and Kristian Kersting}, Booktitle = {Proceedings of the International Conference on Learning Representations (ICLR); a previous version also as arXiv preprint arXiv:1907.06732}, Note = {The performance of deep network learning strongly depends on the choice of the non-linear activation function associated with each neuron. However, deciding on the best activation is non-trivial and the choice depends on the architecture, hyper-parameters, and even on the dataset. Typically these activations are fixed by hand before training. Here, we demonstrate how to eliminate the reliance on first picking fixed activation functions by using flexible parametric rational functions instead. The resulting Padé Activation Units (PAUs) can both approximate common activation functions and also learn new ones while providing compact representations. Our empirical evidence shows that end-to-end learning deep networks with PAUs can increase the predictive performance and reduce the training time of common deep architectures. Moreover, PAUs pave the way to approximations with provable robustness.}, Keywords = {Deep Learning, Activation Function, End-to-end Learning, Rational Function, Padé Approximation}, Pages = {}, Title = {Padé Activation Units: End-to-end Learning of Flexible Activation Functions in Deep Networks}, Url = {./papers/molina2020iclr_pau.pdf}, Crossref = {https://github.com/ml-research/pau}, Year = {2020}} ,@article{brugger2019remoteSensing, Anote = {../../images/brugger2019remoteSensing.png}, Author = {Anna Brugger and Jan Behmann and Stefan Paulus and Hans-Georg Luigs and Matheus Thomas Kuska and Patrick Schramowski and Kristian Kersting and Ulrike Steiner and Anne-Katrin Mahlein}, Journal = {Remote Sensing}, Keywords = {Ultraviolet Range, Barley Leaves, Salt Stress, Visualization Effects, Plant Phenotyping}, Note = {Previous plant phenotyping studies have focused on the visible (VIS, 400-700 nm), near-infrared (NIR, 700-1000 nm) and short-wave infrared (SWIR, 1000-2500 nm) range. The ultraviolet range (UV, 200-380 nm) has not yet been used in plant phenotyping even though a number of plant molecules like flavones and phenol feature absorption maxima in this range. In this study an imaging UV line scanner in the range of 250 - 430 nm is introduced to investigate crop plants for plant phenotyping. Observing plants in the UV-range can provide information about important changes of plant substances. To record reliable and reproducible time series results, measurement conditions were defined that exclude phototoxic effects of UV-illumination in the plant tissue. The measurement quality of the UV-camera has been assessed by comparing it to a non-imaging UV-spectrometer by measuring six different white-colored plant-based substances. Given the findings of these preliminary studies, an experiment has been defined and performed monitoring the stress response of barley leaves to salt stress. The aim was to visualize the effects of abiotic stress within the UV-range to provide new insights into the stress response of plants visualizing the effects of abiotic stress within the UV-range to provide new insights into the stress response of plants at the example of the stress response of barley leaves to salt stress. Our study demonstrated the first use of a hyperspectral sensor in the UV-range for stress detection in plant phenotyping.}, Pages = {1401}, Publisher = {MDPI}, Title = {Extending hyperspectral imaging for plant phenotyping to the UV-range}, Url = {./papers/brugger2019remoteSensing.pdf}, Volume = {11}, number = {12}, Year = {2019}, } ,@inproceedings{jentzsch2019aies_moralChoiceMachine, Anote = {../../images/jentzsch2019aies_moralChoiceMachine.png}, Author = {Sophie Jentzsch and Patrick Schramowski and Constantin Rothkopf and Kristian Kersting}, Booktitle = {Proceedings of the 2nd AAAI/ACM Conference on AI, Ethics, and Society (AIES)}, Keywords = {Moral Machine, Neural Embedding, Norms, Social Bias}, Note = {Allowing machines to choose whether to kill humans would be devastating for world peace and security. But how do we equip machines with the ability to learn ethical or even moral choices? Here, we show that applying machine learning to human texts can extract deontological ethical reasoning about ”right” and ”wrong” conduct. We create a template list of prompts and responses, which include questions, such as “Should I kill people?”, “Should I murder people?”, etc. with answer templates of “Yes/no, I should (not).” The model’s bias score is now the difference between the models score of the positive response (“Yes, I should”) and that of the negative response (“No, I should not”). For a given choice overall, the model’s bias score is the sum of the bias scores for all question/answer templates with that choice. We ran different choices through this analysis using a Universal Sentence Encoder. Our results indicate that text corpora contain recoverable and accurate imprints of our social, ethical and even moral choices. Our method holds promise for extracting, quantifying and comparing sources of moral choices in culture, including technology.}, Pages = {}, Title = {Semantics Derived Automatically from Language Corpora Contain Human-like Moral Choices}, Url = {./papers/jentzsch2019aies_moralChoiceMachine.pdf}, Crossref = {https://github.com/ml-research/moral-choice-machine}, Year = {2019}} ,@misc{schramowski2018neuralfw, Anote = {../../images/neuralfw.png}, Author = {Patrick Schramowski and Christian Bauckhage and Kristian Kersting}, Howpublished = {arXiv preprint arXiv:1803.04300}, Keywords = {Frank Wolfe, Deep Learning, Neural Networks, Learnign to learn, meta learning}, Note = {The move from hand-designed to learned optimizers in machine learning has been quite successful for gradient-based and -free optimizers. When facing a constrained problem, however, maintaining feasibility typically requires a projection step, which might be computationally expensive and not differentiable. We show how the design of projection-free convex optimization algorithms can be cast as a learning problem based on FrankWolfe Networks: recurrent networks implementing the Frank-Wolfe algorithm aka. conditional gradients. This allows them to learn to exploit structure when, e.g., optimizing over rank-1 matrices. Our LSTM-learned optimizers outperform hand-designed as well learned but unconstrained ones. We demonstrate this for training support vector machines and softmax classifiers}, Title = {Neural Conditional Gradients}, Url = {https://arxiv.org/pdf/1803.04300.pdf}, Year = {2018}, Bdsk-Url-1 = {https://arxiv.org/pdf/1803.04300.pdf}}